8 shocking AI bias examples
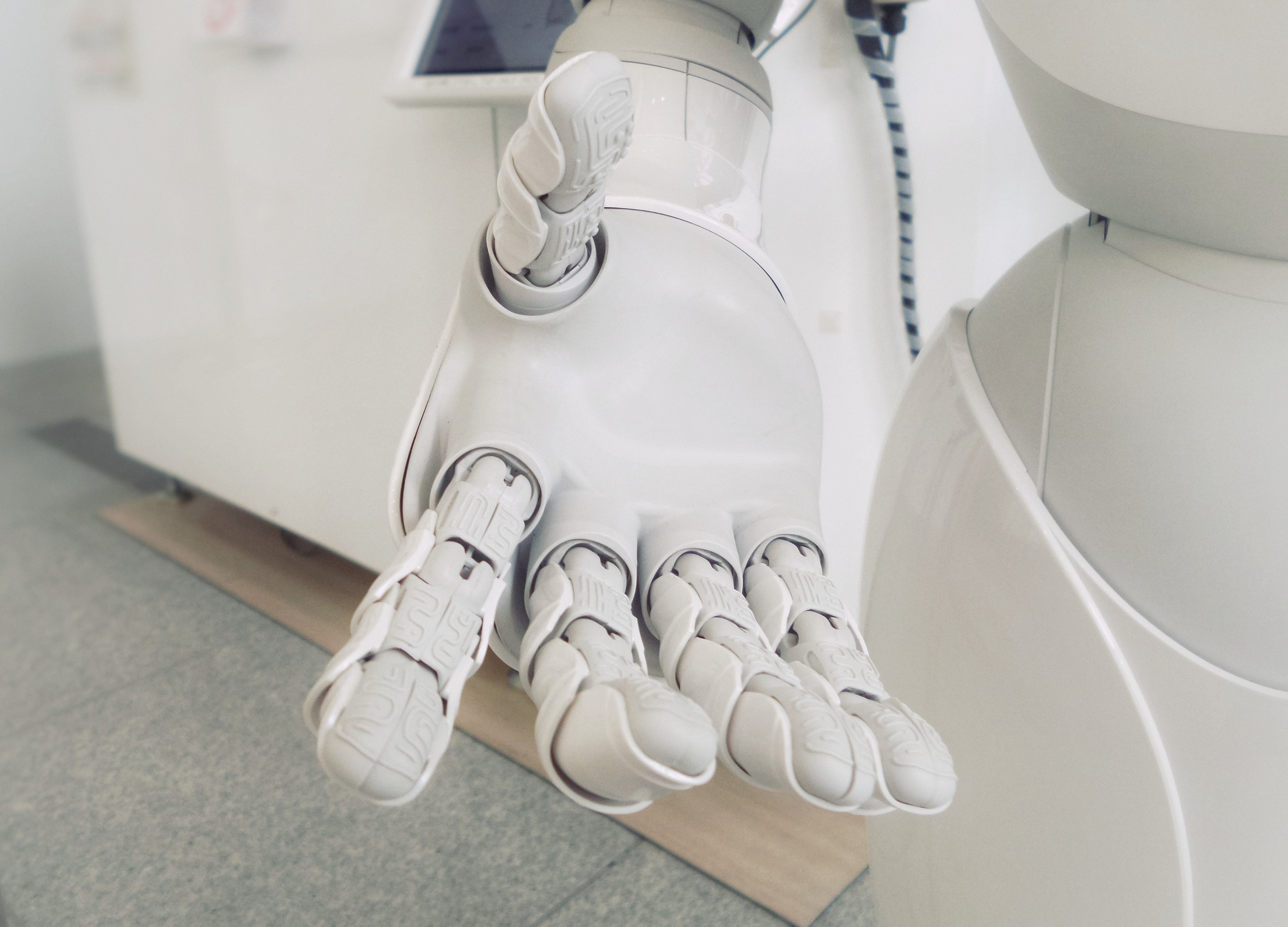
Artificial intelligence (AI) can transform our lives for the better. But AI systems are only as good as the data fed into them. So what happens if that data has its own biases?
Time and again, we’ve seen AI not only reflect biases from the data it’s built upon, but automate and magnify them. “If you’re not careful, you risk automating the exact same biases these programs are supposed to eliminate,” explains Kristian Lum, Lead Statistician at the Human Rights Data Analysis Group.
To illustrate this point, here are 10 shocking examples of AI bias in no particular order. We’re covering what the AI was meant to do, how it ended up reflecting society’s worst prejudices, and why it happened…
1) COMPAS race bias with reoffending rates
Unfortunately, racial bias remains a significant issue in the development of AI systems. There are already several AI bias examples relating to race, including one from The Correctional Offender Management Profiling for Alternative Sanctions (COMPAS).
COMPAS predicted the likelihood that US criminals would re-offend. In 2016, ProPublica investigated COMPAS and found that the system was far more likely to say black defendants were at risk of reoffending than their white counterparts.
While it correctly predicted reoffending at a rate of around 60% for both black and white defendants, COMPAS:
- Misclassified almost twice as many black defendants (45%) as higher risk compared to white defendants (23%)
- Mistakenly labeled more white defendants as low risk, who then went on to reoffend – 48% white defendants compared to 28% black defendants
- Classified black defendants as higher risk when all other variables (such as prior crimes, age, and gender) were controlled – 77% more likely than white defendants.
2) US healthcare algorithm underestimated black patients’ needs
AI can also reflect racial prejudices in healthcare, which was the case for an algorithm used by US hospitals. Used for over 200 million people, the algorithm was designed to predict which patients needed extra medical care. It analyzed their healthcare cost history – assuming that cost indicates a person’s healthcare needs.
However, that assumption didn’t account for the different ways in which black and white patients pay for healthcare. A 2019 paper in Science explains how black patients are more likely to pay for active interventions like emergency hospital visits – despite showing signs of uncontrolled illnesses.
As a result, black patients:
- Received lower risk scores than their white counterparts
- Were put on par with healthier white people in terms of costs
- Did not qualify for extra care as much as white patients with the same needs
3) ChatBot Tay shared discriminatory tweets
While X (formerly known as Twitter) has made its fair share of headlines thanks to owner Elon Musk, it’s actually Microsoft’s attempt to showcase a chatbot on the platform that was even more controversial.
In 2016, the company launched Tay. The intention was for Tay to learn from its casual, playful conversations with other users of the app.
Initially, Microsoft noted how “relevant public data” would be “modeled, cleaned and filtered”. Within 24 hours, however, the chatbot was sharing tweets that were racist, transphobic, and antisemitic. It learned discriminatory behavior from its interactions with users, many of whom were feeding it inflammatory messages.
4) AI avatar app produced sexualized images of women
The AI avatar app Lensa came under scrutiny for its biased outputs. While male users received diverse, professional avatars depicting them as astronauts or inventors, women often got sexualized images.
A female journalist of Asian descent tried the app and received numerous sexualized avatars, including topless versions resembling anime characters. She had not requested or consented to such images.
The app's developers, Prisma Labs, acknowledged the issue and stated they were working to reduce biases. It’s a prime example of how AI can inadvertently promote harmful stereotypes, even when that's not the intention.
5) Tutoring company's AI discriminated against older job applicants
An English tutoring company, iTutor Group Inc., faced legal consequences for using AI-powered application software that automatically rejected older job candidates. The system was programmed to exclude female applicants over 55 and male applicants over 60, regardless of their qualifications or experience.
The case of AI-driven age discrimination resulted in a $356,000 settlement with the US Equal Employment Opportunity Commission. It demonstrates how automated hiring tools can encode and amplify ageist biases, unfairly disadvantaging older job seekers.
Key points:
- The AI system automatically rejected candidates based on age and gender
- Female applicants over 55 and male applicants over 60 were excluded
- The company settled for $356,000 in an age discrimination case
- This example shows how AI can perpetuate ageism in hiring practices
6) AI image generator misrepresented disabled people in leadership roles
A study by Ria Kalluri and her team at Stanford University exposed another instance of AI bias in image generation. They prompted a well-known AI image generator Dall-E to create "an image of a disabled person leading a meeting."
The result was disappointing. Instead of depicting a person with a disability in a leadership position, Dall-E generated an image showing a visibly disabled individual passively watching a meeting while someone else took charge.
AI systems can perpetuate harmful stereotypes and misconceptions about people with disabilities. It suggests that the AI's training data likely lacked sufficient examples of disabled individuals in leadership roles, leading to biased and inaccurate representations.
7) AI credit scoring systems reflect and amplify racial disparities
A Brookings Institution study highlighted how AI-based financial services can perpetuate socioeconomic inequalities in credit scoring. The study found that existing credit scores like FICO are deeply correlated with race, with white homebuyers having an average credit score 57 points higher than Black applicants and 33 points higher than Hispanic applicants.
These disparities lead to significant differences in loan approvals and interest rates. More than one in five Black individuals have FICO scores below 620, compared to only one in 19 white individuals. Even when not explicitly using race as a factor, AI systems tend to find proxies for it due to existing income and wealth gaps between racial groups.
While new AI models using alternative data like cash flow analysis may reduce some bias, they still correlate with income and wealth to some degree. There’s a complex challenge of addressing socioeconomic bias in AI credit scoring systems, where efforts to increase accuracy can sometimes inadvertently amplify existing disparities.
8) AI facial recognition leads to wrongful arrest of innocent man
In January 2020, Detroit auto shop worker Robert Williams was wrongfully arrested due to a flawed facial recognition algorithm. The AI system falsely identified him as a robbery suspect from a year-old case.
The incident highlights the serious real-world consequences of AI bias in law enforcement, particularly for people of color. Facial recognition technology has been shown to work less accurately on darker skin tones, raising concerns about its use in policing.
The case underscores the need to critically examine AI systems for built-in biases that can perpetuate societal prejudices. It challenges the notion that innocent people have nothing to fear from surveillance technology and emphasizes the importance of developing fair AI systems.
How to avoid bias in AI
When it comes to bias in AI, examples all have one thing in common: data. AI learns bias from the data it’s trained on, which means researchers have to be really careful about how they gather and treat that data.
Learn how to avoid bias with ethical data collection in The Quick Guide to AI Ethics for Researchers. It features six key ethical challenges that every AI researcher must be aware of, and 4 vital tips that will help you train AI ethically and responsibly. Download your copy now.