AI data scraping: ethics and data quality challenges
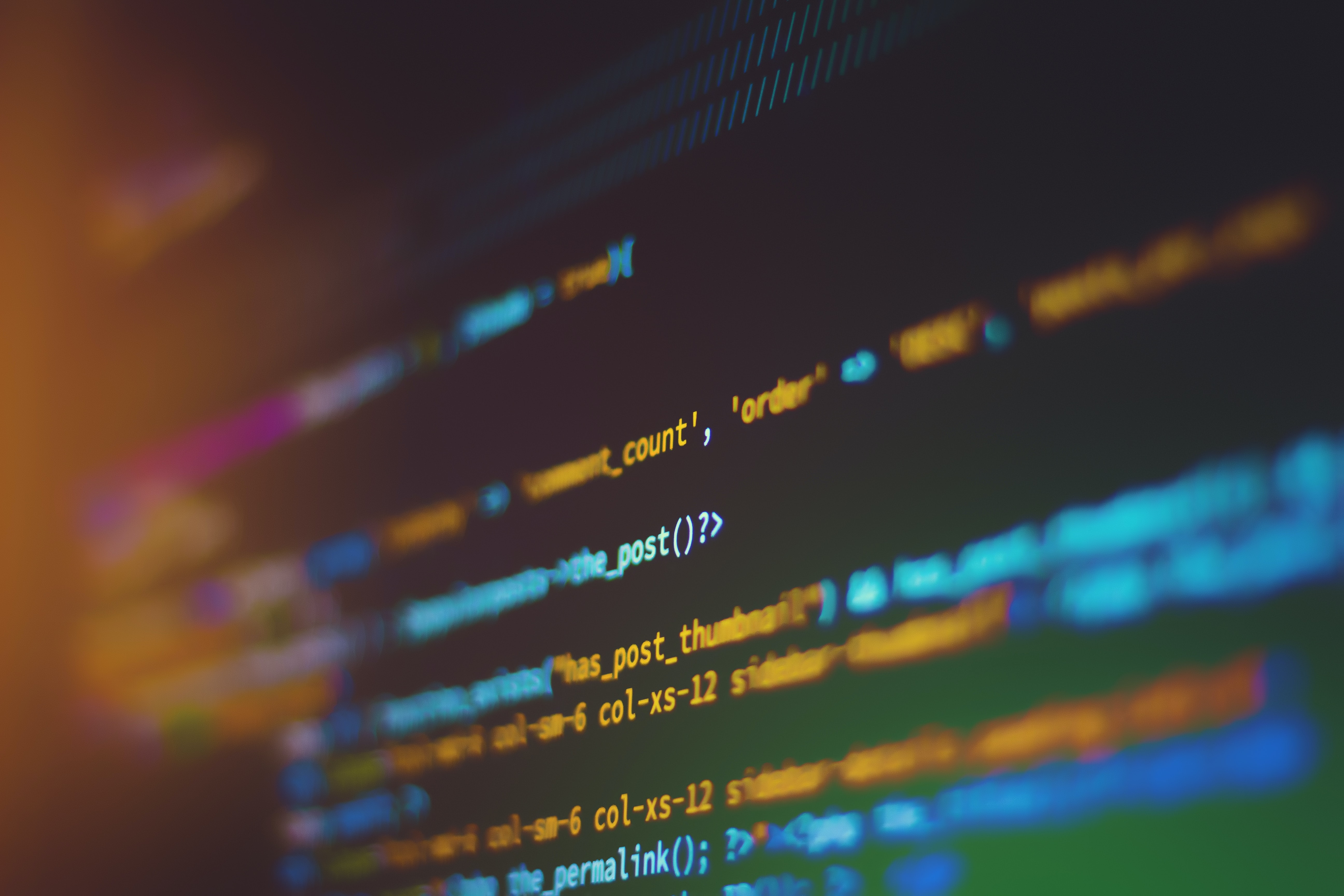
Machine learning models need high-quality training data. To create the desired output, they first need to analyze large amounts of relevant data. AI data scraping is one of the most popular methods for gathering this training data.
However, there are many ethical issues associated with this method. Feeling unsure about the right method to use? In this post, we’ll examine the ethical considerations of data scraping – and consider the benefits of controlled data collection as an alternative.
What is AI data scraping?
Data scraping is the process of automatically extracting data from online sources. These sources include social media pages, video sharing sites and stock image sites. You can use several methods to scrape data, including:
- Manual data scraping – This involves pasting the relevant data into a spreadsheet or document, so it's very time-consuming.
- Automatic data scraping – This is conducted with software or programming scripts. These include web crawlers or scraping libraries.
AI researchers need a considerable amount of data to work with. While AI data scraping can help with this, web users have raised ethical concerns - like sources being scraped without the creator's consent.
The ethical challenges of AI data scraping
There's no question that AI can generate impressive output. But it can only do this after being fed large amounts of data. Data scraping can gather billions of pieces of data automatically for use in AI training. But where does this data come from?
It’s an important question. And it’s where the ethical issues with AI data scraping arise, whether text, image, video or audio (multimodal). Some of the main issues to be aware of are...
Accountability
Many issues relating to AI data scraping relate to image and video content. A key example of this is Stable Diffusion, a well-known AI text-to-image generator from Stability AI. The huge datasets used to train the AI came from a non-profit organization, LAION. Stability AI has provided funding to this organization for compute resources.
In an examination of 12 million images used by LAION, Andy Baio and Simon Willison found that over a million images came from Pinterest. 819k images were scraped from WordPress blogs, 121k from Flickr, and 67k from DeviantArt. The dataset also frequently scraped from ecommerce and stock image sites. Images from living artists are also included.
But how is this possible? Isn’t the content within these datasets protected by copyright law? Well, this is a grey area. There's no strict guidance about using copyrighted material to train AI systems. Whether it's a form of copyright infringement or not is unclear. Still, a much stronger legal defense can be made for using the data outside the commercial sector. Fair use protections are more likely to apply to non-commercial usage. This includes for university or non-profit research.
Here's how it works. Non-commercial organizations create the datasets, as they're protected by fair use. Commercial companies then use their datasets for AI training without repercussions. As Andy Baio of Waxy.org writes, this is an “academic-to-commercial pipeline” that allows companies to avoid accountability for laundering data.
Consent
When creating scraped datasets, many researchers have not asked the original creators for consent. Creators may have consented for their work to be used for non-commercial purposes. But, as we have seen above, much of this data has ended up in the hands of commercial companies.
For example, the MegaFace dataset contained 3.5 million photos with faces scaled from contributors on Flickr. According to Waxy.org, researchers “redistributed it to thousands of groups, including corporations, military agencies, and law enforcement.” Contributors were never asked to opt-in to having their content used in this way. They were not asked to provide informed consent. Even outside of consent concerns, harvesting the internet for data remains ethically questionable. Harmful data, including abusive language or violent content, may be included within the scraped data, for example.
What's more, many AI projects have replicated discrimination and bias in their output. Online creators have raised concerns about their content being used to develop biases in machine learning. Speaking in ARTnews, writer Maya Kotomori shared her experience of uploading selfies to the AI photo editing app, Lensa. Although Kotomori is a light-skinned black person, the first selfies she received from Lensa made her look like a white woman. After repeating the process, she raised concerns about how her selfies had potentially been used to “teach” the AI about racial nuance. “How can this help/hurt society in the long run?” she wrote. “The answer is: I have absolutely no idea.”
Attribution
Another ethical issue associated with AI data scraping is creator attribution. For AI to produce accurate, high-quality art, it must first be “trained” by real artwork. Unfortunately, this has led to real artists having their intellectual property stolen as part of data scraping. Some text-to-image AI websites enable commercial companies to generate art in the style of real artists, both living and dead.
Consumers can generate AI images in the style of the artist. Yet the artist will not be attributed. They also won't receive any royalties for the images produced. As consumers can produce these artworks cheaply, this has a direct impact on the earnings of real-life content creators.
This ethical concern extends outside of the art world. From social media posts to online videos, data scraping of everyday content can be used to make money for commercial companies.
Why Prolific’s controlled data collection is better
Fortunately, AI data scraping isn’t the only way to gather data for machine learning.
At Prolific, we don't scrape content from non-consenting online creators. Instead, we provide data from our vetted collection of professional participants. All our participants are fairly compensated for their time and effort. Our platform features a minimum pay level of £6 per hour and a recommended pay level of £9 per hour.
Not only is this method more ethical, but it also provides the highest-quality data. You’ll also only receive data that is relevant to your research question. In this sense, you can think of Prolific as the online version of a sterilized, controlled lab environment. Meanwhile, data scraping remains at risk from outside contaminants. These include discriminatory biases against marginalized groups, harmful language, and graphic content. Controlled data collection is a win-win for ethics and data quality.
High-quality data for AI training
At Prolific, research ethics are a key priority. There are many reasons to seek ethical AI data for machine learning. Aside from fair pay, participants can opt-in to research projects based on their needs. With the option to message researchers and Prolific’s support team, they also have a voice to raise concerns. This ensures researchers get the highest quality data. Participants can be trained to give better data over time, whilst scraping only takes random data from a non-research context. With over 130,000+ vetted participants on our platform, obtaining fast, scalable data doesn’t have to come at an ethical cost.