Anthropomorphic behaviors in large language models: how Prolific enabled a complex quantitative study
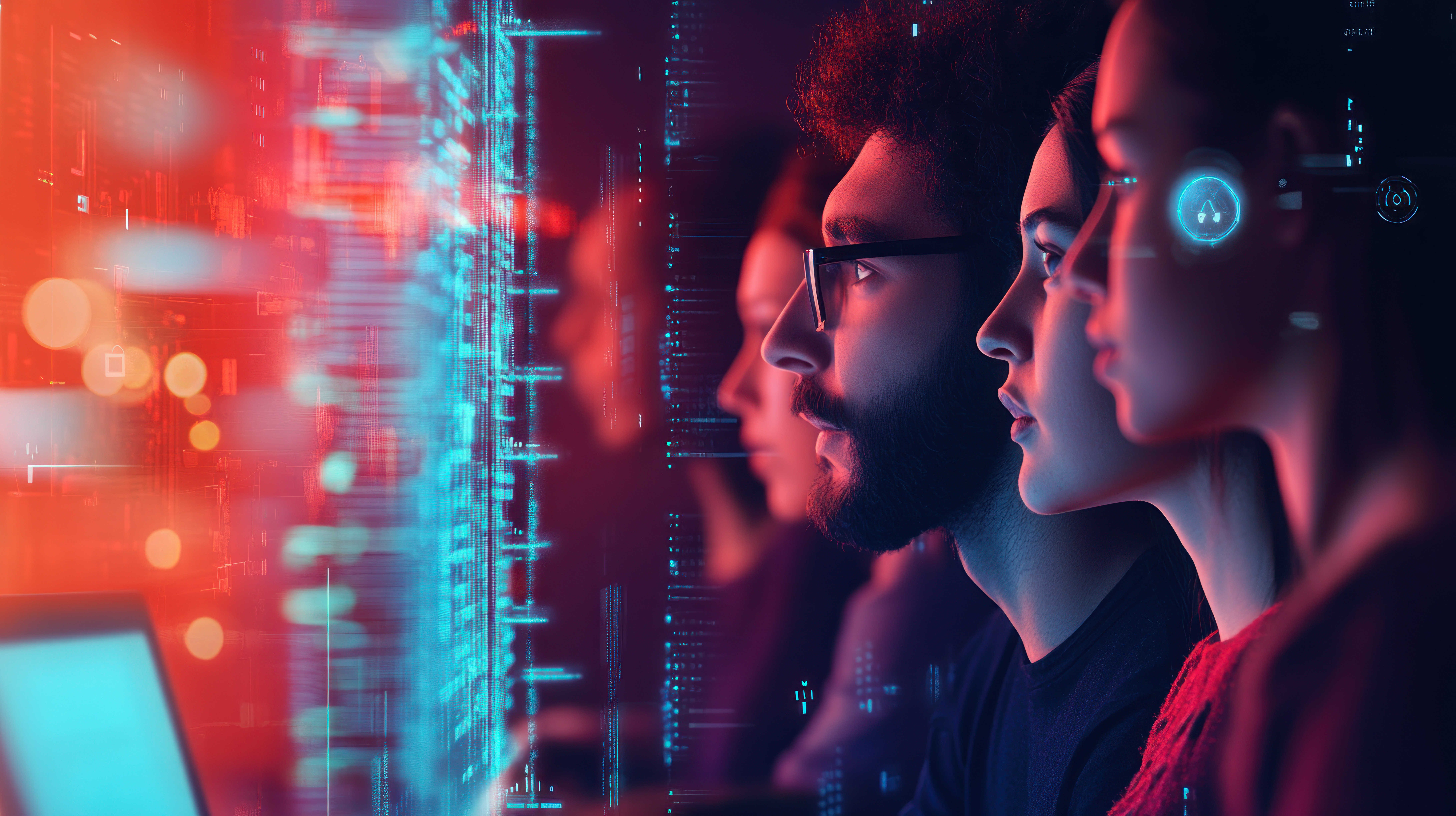
Anthropomorphic behaviors in large language models: how Prolific enabled a complex quantitative study
The challenge
Using large language models (LLMs) can be an uncanny experience. As you ‘chat’ with these systems, it sometimes feels as if you’re conversing with another human. This humanness helps make LLMs engaging and easy to use. It also raises practical and ethical concerns.
In 2024, a research team at Oxford University’s Oxford Internet Institute (OII) developed an evaluation method that could identify anthropomorphic behaviors among LLMs. The research team, however, needed to make sure that their evaluation method corresponded with end users’ perceptions of human-like behavior. To gather this data, the team needed to:
- Recruit a large number of participants
- Invite them to interact with an LLM
- Complete a survey on their perceptions of the humanness of the LLM
The OII’s evaluation method
A common phenomenon among users of LLMs is a tendency to anthropomorphize the systems, projecting human qualities onto the chatbots they interact with. LLMs use various techniques that can create this impression.
The OII researchers wanted to find out exactly how LLMs create the perception of humanness so it could help to develop a method for evaluating LLMs’ anthropomorphic behavior.
The first stage of the research involved assessing the language used by four leading LLMs during ‘conversations’ about various personal topics. After analyzing the language used by the LLMs, the researchers were able to identify 14 kinds of behavior that contribute to perceptions of anthropomorphism (including personhood claims, use of first-person pronouns, or expressions of internal states).
In the second phase, the researchers used a version of the Gemini 1.5 Pro LLM to create two chatbots. The first chatbot was instructed to use highly anthropomorphic behavior (that is, by drawing on the 14 categories identified by the team). The second chatbot was instructed to use much less of this anthropomorphic behavior.
In the experiment, participants were invited to ‘chat’ with one or other of the chatbots for 10-20 minutes. They were then asked to answer a survey about how they perceived the LLM they chatted with.
The solution
The OII researchers needed to recruit a large number of people to participate in the quantitative study. Prolific supported the research by providing:
A large, representative sample
The OII team used Prolific to rapidly recruit 1,101 adult English speakers with an even gender split (49% female, 51% male) and a broad range of age groups (18-90). This ensured the sample was representative and would include a variety of perspectives on how ‘human’ the LLMs appeared.
Thoughtful inputs
Prolific’s members have a reputation for providing thoughtful free text responses. This was necessary for the study since respondents were asked to write a paragraph reflecting on their perceptions of the LLM they interacted with.
LLM experience
Many Prolific members have prior experience of using LLMs and know how to interact with the technology. Their familiarity proved valuable for the researchers, as minimal instruction was required during the experimental stage when participants engaged with the LLM.
The results
As the researchers had hypothesized, the LLM, which used more anthropomorphic language and behaviors, was perceived as more human-like by participants.
This finding is interesting in itself. But it also provides a potentially valuable tool for future studies. The analysis shows that there are 14 categories of behavior that create an impression of humanness. Researchers can therefore use this evaluation model to analyse LLM responses to gauge how end users might perceive them.
If an LLM uses these 14 categories of behavior extensively, it’s more likely that users will project human qualities onto the system. If an LLM minimizes the use of this behavior, users may be more likely to perceive the system as ‘just’ an AI.
Conclusion
Prolific played a key role in the study, providing the OII team with a large, diverse and engaged participant pool. We offer a highly effective way of gathering reliable, high-quality data, as well as detailed, thoughtful responses from hundreds or even thousands of respondents.
Learn more about how Prolific can support your research here.
Citation: Ibrahim, L. et al, (2025), Multi-turn Evaluation of Anthropomorphic Behaviours in Large Language Models: https://arxiv.org/pdf/2502.07077
Research institutions: The University of Oxford, Oxford Internet Institute