Everything you need to know about data annotation jobs
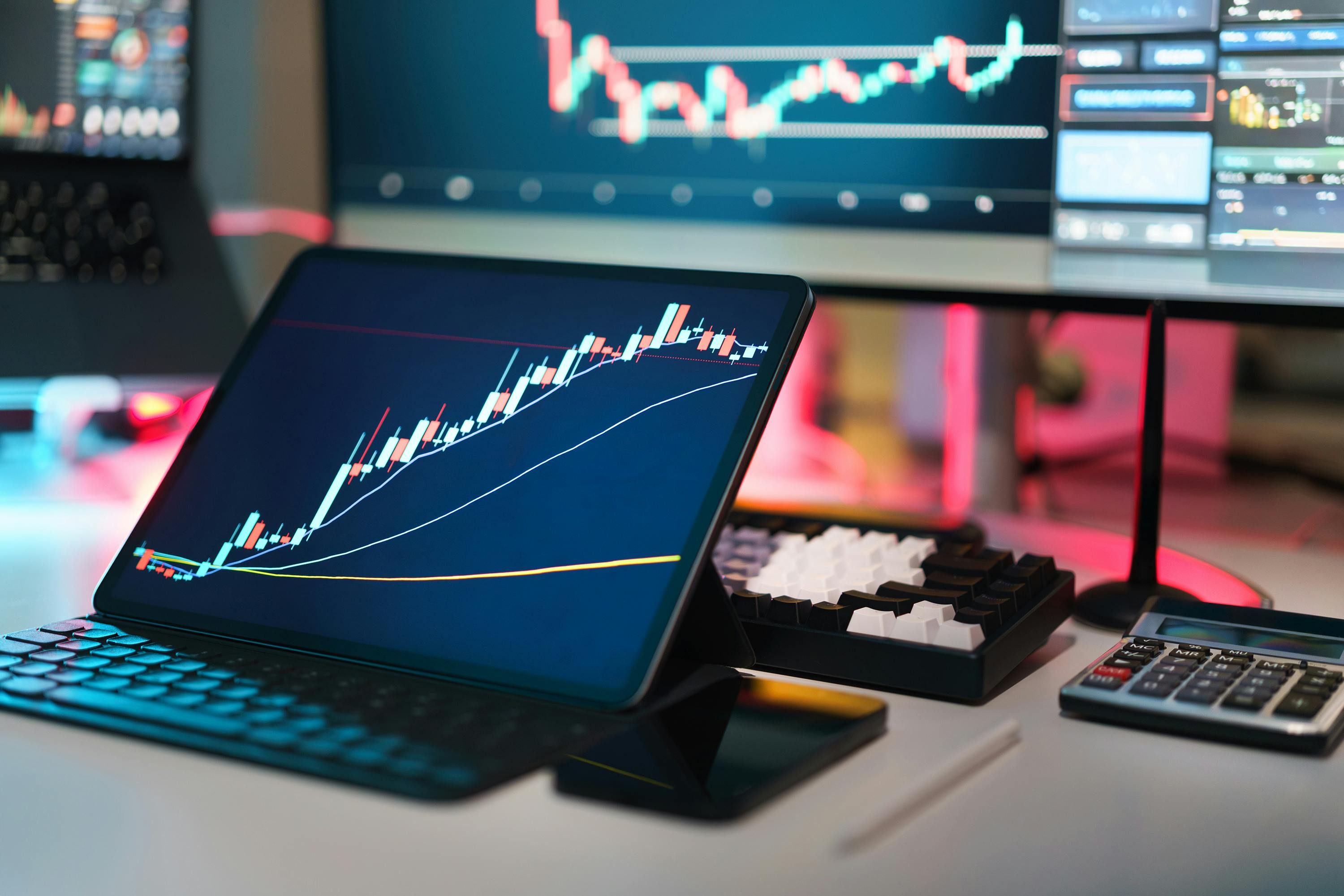
As AI systems become more sophisticated, the need for human feedback grows all the more important. Data annotation jobs, sometimes referred to as AI annotation jobs, play a central role here, improving how AI works— from making chatbots more helpful to ensuring image recognition is accurate. Whether you're curious about getting involved or looking to understand the field better, here's a complete guide to data annotation work.
Data annotation in a nutshell
Data annotation is the process of reviewing and providing structured feedback on AI outputs. When AI systems generate text, images, video, or engage in conversations, they need human input to understand if they're performing well.
That’s where, you guessed it, humans come in. The work varies widely. You might check if AI-written text sounds natural, verify whether AI-generated images match their descriptions, or test if chatbots maintain sensible conversations. Providing human feedback helps researchers identify where their systems need improvement and confirms when changes actually make things better.
Types of data annotation work
Data annotation tasks generally fall into several main categories, each focused on different aspects of AI performance.
- Text annotation involves reviewing written content, from short responses to longer documents, checking for accuracy, clarity, and natural language. Image annotation ranges from basic object identification to evaluating complex AI-generated artwork.
- Conversation evaluation tests how well AI systems maintain dialogue, often through extended back-and-forth interactions.
- Safety testing focuses on identifying potential issues or inappropriate content.
- Some specialized tasks need specific expertise, like technical documentation review or code evaluation.
The role of RLHF
RLHF (Reinforcement Learning from Human Feedback) might sound technical, but it's simply about AI systems learning from people's responses. Every time participants provide feedback—whether rating chatbot responses or checking AI-generated images—they're helping AI understand what works and what doesn't.
If someone rates whether an AI response was helpful or marked if an image matched what was requested, that feedback, combined with input from many other participants, helps researchers guide their AI systems toward better performance. It's a straightforward way of showing AI the difference between good and not-so-good outputs.
Learn more about the different types of data annotation jobs
Who does data annotation work?
Data annotation brings together a diverse community of participants. Some people focus on general feedback tasks that benefit from everyday user perspectives, like rating how natural AI conversations sound or checking if AI-generated images match descriptions. Others bring specialized knowledge to technical tasks, such as reviewing AI-generated code or evaluating scientific content.
What's interesting is the variety of backgrounds involved. Students, professionals, retirees, and people from all walks of life contribute their perspectives. Diversity matters because AI systems need feedback from many different viewpoints to work well for everyone.
How annotation tasks work
Most annotation tasks follow a straightforward process. You'll receive AI-generated content along with specific guidelines about what to evaluate. This could be anything from rating how well an AI response answers a question to comparing different versions of AI-generated images.
Quality matters more than speed. Tasks often include test questions to ensure reliable data, and researchers look for consistent, thoughtful feedback. While some tasks need quick decisions, others require detailed explanations about what works or needs improvement.
Learn more about how data annotation jobs work
Getting started with annotation work
You don't usually need specialized knowledge to begin, as many tasks just need clear thinking and attention to detail. More technical tasks might need specific expertise, but there's plenty of work reviewing everyday interactions like chatbot conversations or image generation.
Pay rates vary based on task complexity and time requirements. Simple tasks might take minutes, while detailed evaluations can run longer. At Prolific, researchers set rates starting at $20 (£16) to $50 (£40) per hour, with higher pay often offered for specialized knowledge or complex tasks.
What makes good data annotation work?
Success in data annotation comes down to consistency and attention to detail. Good annotators maintain the same standards throughout their work, whether they're rating the first or fiftieth AI response. They focus specifically on what researchers have asked them to evaluate, rather than getting sidetracked by other aspects of the AI's performance.
Clear feedback makes a real difference. Instead of vague comments like "this doesn't work," helpful feedback points out specific issues: "the AI's response introduces new topics without addressing the original question." Precise input will help researchers understand exactly what needs improvement.
The future of data annotation
As AI systems become more complex, the need for human feedback grows. New types of annotation work emerge as AI tackles more sophisticated tasks, from creative writing to complex problem-solving. This creates opportunities not just for general feedback, but for people with expertise in specific fields.
The field keeps evolving as AI capabilities expand. What started with basic image labeling has grown into nuanced evaluation of AI performance across many areas. For anyone interested in shaping how AI develops, data annotation offers a direct way to influence these important technologies.
Getting involved with data annotation jobs
Data annotation offers a unique opportunity to contribute to the development of AI technology. As these systems become more integrated into daily life, the need for thoughtful human feedback only increases. Whether you're interested in general evaluation tasks or have specific expertise to share, there are plenty of ways to get involved.
Data annotation jobs with Prolific
Prolific connects independent participants with researchers running data annotation studies. Researchers post a variety of tasks, from quick evaluations to in-depth testing sessions.
Your responses help make AI systems more accurate and reliable. Unlike repetitive data labeling work, these tasks often involve giving detailed opinions and testing how AI systems handle real-world situations.
You can browse available studies and choose ones that match your interests and schedule. Your feedback helps shape how AI systems develop, making them more effective and reliable for everyone.