How Carnegie Mellon University used Prolific to create a dataset for text-to-image AI models

Imagine a world where AI doesn't replace artists, but enhances their creativity. That's the scenario researchers at Carnegie Mellon are exploring. They looked at how illustrators and designers interact with AI tools like text-to-image generators, aiming to understand the skills needed for effective co-creation.
Using Prolific's platform to connect with professionals, the team gathered valuable insights into this emerging creative dynamic. Their findings could shape how we approach AI integration in creative fields.
The goal of the research
Frederic Gmeiner, a PhD researcher at Carnegie Mellon’s Human-Computer Interaction Institute, is spearheading this innovative work. His goal? Build a first-of-its-kind dataset capturing professionals’ real experiences co-creating with text-to-image AI.
“We are observing increasingly generative AI systems with the potential to enhance professional workflows,” Gmeiner says. “But we also notice professionals often struggle with learning to work with these systems effectively.”
By studying professional illustrators using AI for open-ended creative tasks, Gmeiner wanted to understand the skills required to use AI as a true partner rather than a tool. To achieve this, the team covered:
- Learning strategies
- Prompting techniques
- Decision-making processes
- Conceptual skills
The research’s aim was to answer fundamental questions about the interaction dynamics between humans and AI. This is especially true for how professional illustrators can integrate AI into their creative process without losing their unique artistic touch.
Understanding these dynamics is fundamental. It will help in designing AI systems that are not only more intuitive but also better aligned with the professional standards and expectations of human creators.
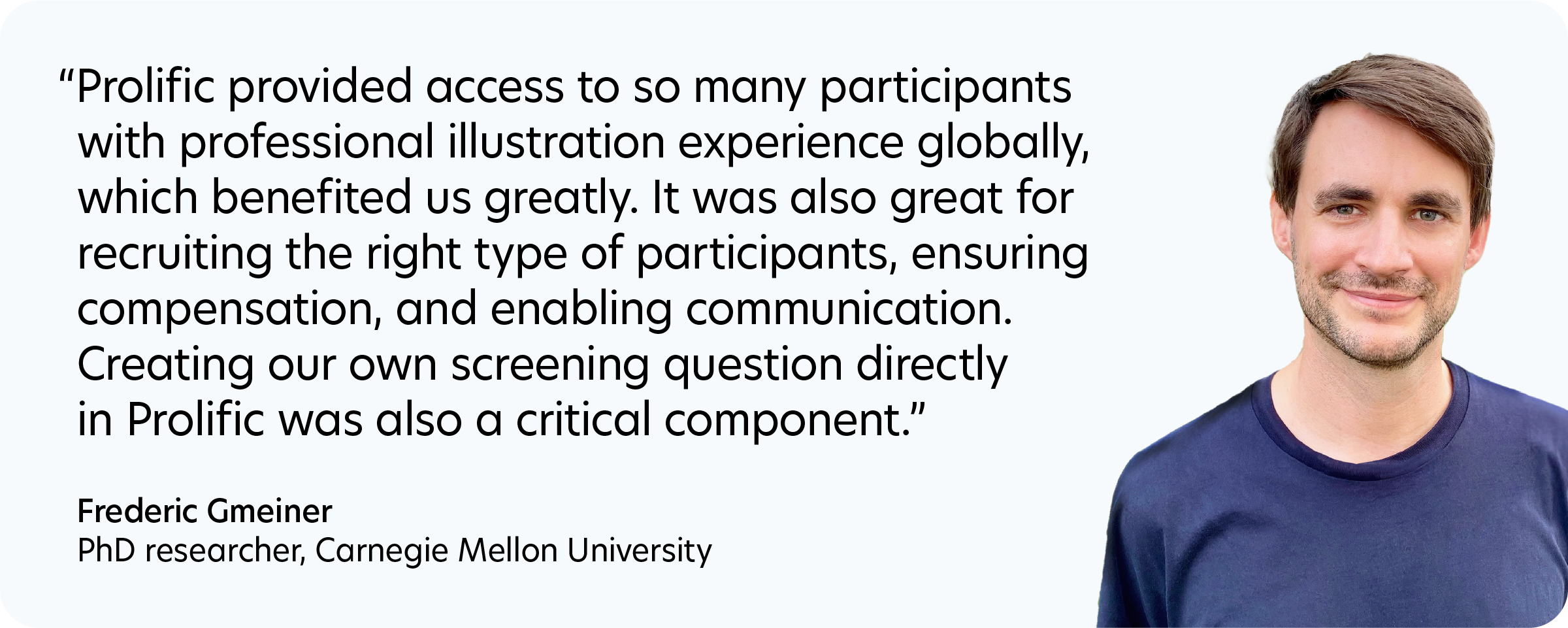
The challenges researchers faced
But achieving this goal meant overcoming three major hurdles.
Recruitment
First was recruiting professional illustrators to participate—not just any creative professionals, but active experts with real illustration production experience.
Designing the right interface
The second challenge? Designing an experimental interface mimicking real creative workflows while allowing systematic data collection on all behaviors. Think text prompts, AI outputs, audio and visuals, participant interactions, and more.
This interface needed to be strong enough to handle the complexities of creative tasks. But it also had to be user-friendly to encourage natural interaction.
Sophisticated data collection
Finally, Gmeiner’s team required custom data collection software. It needed to be sophisticated enough to accurately log the rich behavioral streams of professionals as they prompted AI assistants throughout the creative process.
The software had to integrate effortlessly with the experimental interface, so that all relevant data points were captured without interrupting the creative flow of the participants. Additionally, the team had to guarantee data security and privacy. They were dealing with personal creative works and potentially sensitive information.
Watch the full video case study.
The solution with Prolific
Gmeiner turned to Prolific’s advanced capabilities to overcome these obstacles. Prolific’s powerful pre-screening meant reaching qualified professional illustrators active across the likes of illustration for publications, media, and advertising.
“Prolific provided access to so many participants with professional illustration experience globally,” Gmeiner says. “This benefited us greatly.
“It was also great for recruiting the right type of participants, ensuring compensation, and enabling communication,” Gmeiner adds. “Creating our own screening question directly in Prolific was also a critical component.”
With Prolific handling participant sourcing, Gmeiner’s team built custom web software facilitating AI collaboration while capturing comprehensive multimedia logs as data. The approach allowed the research team to focus on refining the AI interaction models and analyzing the rich datasets generated by the participants.
Prolific’s streamlined participant management process meant the researchers could achieve a high level of engagement and satisfaction among participants. This was necessary for obtaining high-quality data.
Why Prolific?
While developing novel AI software in-house, Prolific streamlined all other operational aspects—recruitment, vetting, secure participant access, and engagement across different study stages.
“We used Prolific’s built-in pre-screening which was awesome,” Gmeiner says. “But Prolific also directly added our specialized illustration experience question tremendously aiding recruitment.”
Targeted recruitment ensured that only participants with the requisite skills and experience were included, thereby enhancing the validity and reliability of the study results. Moreover, Prolific’s platform facilitated continuous communication with participants. This was essential for addressing any issues promptly and maintaining a smooth workflow throughout the study period.

The results and key findings
Over 90 global professional illustrators completed Gmeiner’s text-to-image AI co-creation tasks using the custom web app. It generated unprecedented qualitative datasets with comprehensive multimedia logs documenting:
- Full prompt sequences and AI interaction histories
- Iterative prompt refinements based on AI outputs
- Real-time ratings and qualitative feedback
- System messages or AI suggestions received
- Final creative assets and survey responses
"We have all the details—interaction logs, recordings, generated assets," Gmeiner says. "It provides full visibility into their prompted AI collaboration process."
The detailed logs offer insights into how professionals adapt their strategies over time, what kinds of prompts lead to successful outcomes, and how they respond to AI-generated suggestions.
These insights are invaluable for understanding the learning curve associated with using AI tools in a professional context. They can inform the development of more intuitive and effective AI systems.
Gmeiner's team already published groundbreaking findings on how interventions impact professionals' ability to recognize AI model limitations, a key skill for effective co-creation.
- The guided exploration condition had a positive effect on participants' ability to identify AI model limitations.
- This effect was particularly strong for participants who initially had lower pre-test scores in identifying these limitations.
- The ability to identify model limitations was classified as a conceptual skill important for learning to co-create with generative AI systems.
- The research demonstrated that it's possible to measure improvement in this skill before and after interacting with the AI system.
- This approach provided a way to evaluate different support mechanisms for human-AI co-creation in open-ended task domains.
- The findings suggest the importance of designing AI systems that are transparent and provide meaningful feedback to users.
- While identifying model limitations is an important skill, the researchers acknowledge it's likely not the only important skill for effective human-AI co-creation.
By identifying common pitfalls and effective strategies, the research paves the way for developing educational resources and training programs that can help professionals integrate AI into their workflows more seamlessly.
What comes next?
Looking ahead, this unique illustrator data represents "a goldmine" for further revelations optimizing:
- Human-AI creative workflows
- Educational frameworks
- Prompt strategies
- More ethical, seamless collaboration paradigms
As AI continues to evolve, ongoing research will be central for ensuring that these tools enhance rather than hinder creative processes. The goal is to maintain the delicate balance between human intuition and machine efficiency.
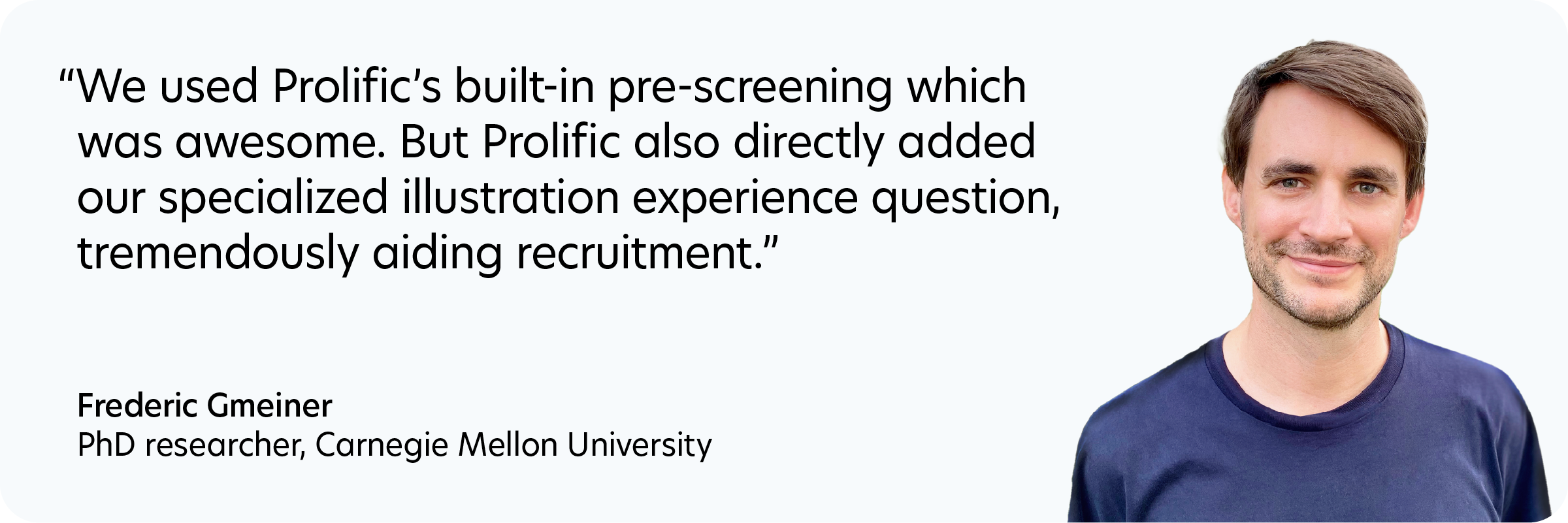
Summary: the future of human-AI creative collaboration
As AI capabilities grow, Gmeiner’s pioneering work is helping elevate the voices and experiences of real creative professionals. It paves vital paths toward intuitive human-machine creative partnerships.
Through rigorous qualitative study, Carnegie Mellon is illuminating how AI can become an extension of human creativity and cognition. This research not only advances our understanding of human-AI interaction but also sets the stage for developing the next generation of AI tools that can truly co-create with humans, enhancing creativity and productivity across various professional fields.
Find out more in the full Carnegie Mellon video case study.
Need to find vetted research participants at scale, and fast? Get started for free with Prolific.