How Clemson University used Prolific to explore human-AI collaboration

Dr Christopher Flathmann is a research assistant professor, working closely with Dr Nathan McNeese in human-centred computing at Clemson University. Together they head Clemson’s TRACE (Team Research Analytics in Computational Environments) research group as associate director and director, respectively.
Here, they explore the acceptance of human-AI teamwork and aim to advance the design and build trust in AI systems acting in team environments.
Part of this is looking at the responsible creation of AI teammates and how these teammates can be valuable in making people’s lives easier by reducing cognitive workload. For example Dr. Flathmann and Dr. McNeese have worked to inform the use of AI teammates in extreme environments underwater or near high radiation and even in educational settings where AI can help teachers plan lessons and learn new information.
Their recent study focused on how language used to describe AI can influence people’s perception of AI, and whether humans see it as an innocuous tool or whether they could view it as a highly functional teammate.
Prolific proved to be invaluable to this research, combining diverse data sets with real-time usage reports to provide accurate results.
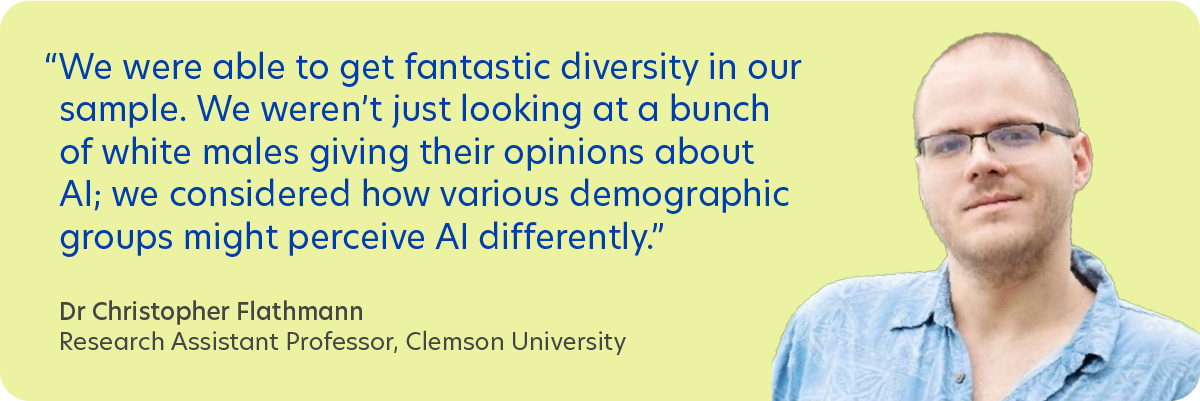
The task
In Dr Flathmann’s own words, the team investigates “how to best design AI and train humans to work independently with each other.”
The concept of humans working with AI teammates is fairly new. So, for the last couple of years, Dr Flathmann and Dr McNeese have been focusing on precisely what AI, in this case, a Large Language Model (LLM), needs to accomplish to be viewed as a team member.
TRACE wanted to show how an LLM is perceived by its human user, and whether this response is affected by how it’s presented – specifically, whether using the descriptor “teammate” vs. “tool” can alter perception.
A prior project showed that the word “teammate” created negative expectations and even questions of job security from respondents. These expectations, though, were rooted only in speculation.
Now that people are using and interacting with AI systems more regularly, the next logical step was for TRACE to focus on practical interaction. This way they could see whether findings support these negative expectations.
Sign up to watch the full video case study
The challenge
Any finding based on public perception must also recognise the reality that each individual is different and can respond differently to AI.
Designing one system to fit every difference is complex, and including every single difference could result in false noise. They therefore needed to identify which parameters would be impactful and focus their attention on those.
TRACE needed a way to ensure their participants were representative of the public, based on the most impactful demographics, to ensure appropriate and accurate results.
This style of research could typically take months, though, so they looked for a way to speed things up without sacrificing quality.
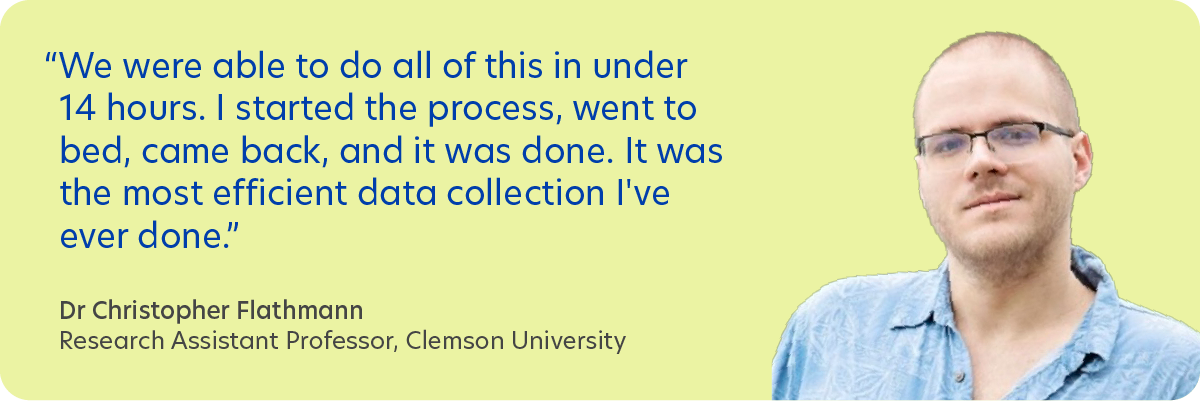
The solution
Dr Flathmann found Prolific to be the exact solution they needed to form their novel data set.
He states: “We were able to get fantastic diversity in our sample. We weren’t just looking at a bunch of white males giving their opinions about AI; we considered how various demographic groups might perceive AI differently.”
Prolific’s platform holds a diverse pool of possible participants. And detailed demographic filters allowed them to select a demographic that represented the population of the USA based on age, gender, and race.
And though the sample size of 778 may not seem large in some domains, Dr Flathmann comments that it can prove difficult to find even 100 participants for their specific research. Having almost 800 people with this level of diversity was fantastic for the study.
But a study needs more than participants.
To implement their research, TRACE needed a way to monitor practical interactions so they could analyse how people viewed the LLM in real-time.
The team combined:
- Prolific as the recruiting platform,
- Qualtrics to create the survey, and
- OpenAI ChatGPT3.5 Turbo as the LLM.
It began with a survey through Qualtrics, distributed to participants by Prolific, integrated with Chat GPT3.5 Turbo and JavaScript for simple data extraction.
Participants were asked to perform a task that could easily fall into the AI use category of tool or teammate, namely travel planning, so as not to confuse results.
Each time the participant sent a message, the AI response would say “tool” or “teammate” and then display the LLM’s reply. Functionally, it acted in the same way for both groups of participants. The only differences were this one simple descriptor and any resulting changes in human perception and behavior.
In a short 5-minute conversation, the team was able to look at messaging between ChatGPT and the human participant, analysing the semantics for positive, neutral, or negative language.
While this type of research could take months, with Prolific it was complete in under 14 hours.
Dr Flathmann notes, “We were able to do all of this in under 14 hours. I started the process, went to bed, came back, and it was done. It was the most efficient data collection I've ever done.”

The results
While Dr Flathmann and Dr McNeese await the lengthy publication process, they can share some findings – and it’s not quite what they expected.
The data suggests that participants, surprisingly, communicated more positively with AI when it was marked as a teammate rather than a tool.
“This was a landmark finding for our group,” says Dr Flathmann, describing the very first time research has shown a positive response when referring to an AI as a teammate.
Most notably, the language used towards the LLM was more positive, demonstrating a stronger connection and greater trust when viewed as more than a mindless tool.
The surprising findings now open up a whole new branch of improvements to the way AI functions, charting the course for more positive human engagement. Dr Flathmann posits, “Maybe for a future study we could look at how we can maximize that positive perception. Can we widen that gap?”
The successful combination of Prolific, Qualtrics, and ChatGPT3.5 Turbo has proven that this type of research is not just possible, it’s efficient, quick, and enlightening.
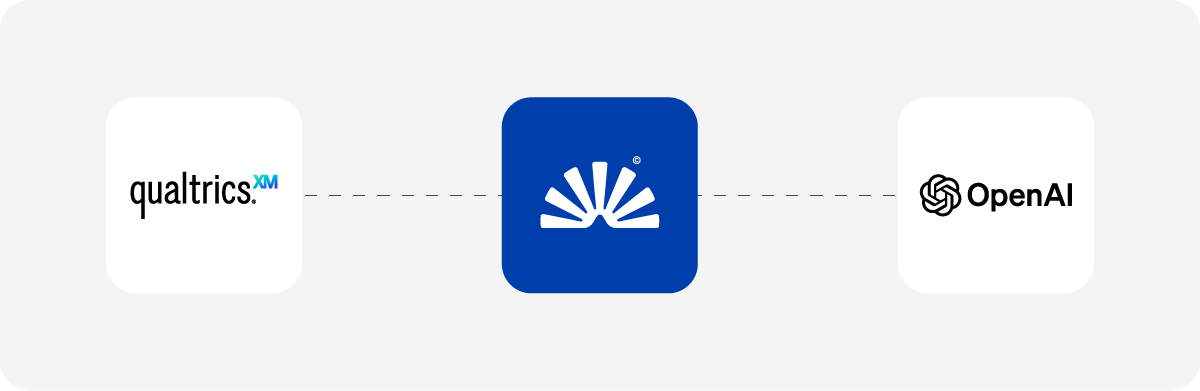
A bright future for human-AI collaboration
Paving the way for future research, it’s exciting times ahead for TRACE and the improvement of human-AI engagement.
Using Prolific to access an advanced data set, they’ve been able to quickly find fascinating results that could shape a more harmonious future for human-AI relationships.
Find out more in the full Clemson video case study.
Need to find vetted research participants at scale, and fast? Get started for free with Prolific.