How Prolific enabled Layer 6 to expose flaws in generative model evaluation metrics

Layer 6 AI develops machine learning systems that advance the field of AI and have a positive real-world impact. The team at Layer 6 conducts regular research into how AI is used in familiar areas like banking and healthcare.
Part of generative AI development is measuring how realistic it is or at least perceived to be. If you want AI-generated images to be indistinguishable from “real” images, for example, you need to continually assess the models and their outputs with state-of-the-art evaluation systems.
But what if the metrics used to evaluate them are flawed? Anthony Caterini, Senior Machine Learning Scientist at Layer 6, and the team decided to find out. By identifying and exposing these flaws, they aimed to improve future evaluation methods - and the models themselves.
Their recent paper “Exposing flaws of generative model evaluation metrics and their unfair treatment of diffusion models” details this research, which compares human evaluation to AI evaluations and aims to highlight any discrepancies.
Layer 6 chose Prolific to provide a crucial cog in the research machine – a comprehensive human participant data set.
The task
Caterini explains, “We started to notice a difference between what humans perceive as high-quality images and what people are actually using to create their images.” This discrepancy suggested that there was a problem. Not necessarily with the models themselves, but with the traditional evaluation metrics.
Evaluating the images involved measuring the distance between summary statistics in a specific embedding system, rather than measuring pixel space or comparing the images directly. The process was done in a closed loop with no human interaction: the metrics were calculated, and the performance was reported.
Caterini and his team noticed something. When this embedding system was similar to the model that was used to generate the image, the metric was biased, and the model scored highly. But in actual fact, people were using a different type of model (diffusion) to generate their images.
To investigate their hypothesis, they’d need to involve real people in the evaluation process. That way, they’d discover if an evaluation system can rate generated image performance accurately - as a real person would.
The challenge
The team established that images generated by models sharing similarities with the evaluation framework would score more highly. So, they studied alternative evaluation frameworks based on different embedding models for comparison.
Alternative models selected, the team turned their attention to the crux of the matter – real people. Layer 6 needed participants to provide the baseline, but not just anyone would do. They needed a large base of vetted evaluators from a reliable source. They also wanted to make sure each participant was fluent in English and well-educated so the study could take place seamlessly.
The solution
Caterini and his team implemented a “two-alternative forced choice test to show that the metrics were flawed.” As people don’t have the statistical affinity that an evaluation model could, the participants began with a shorter training phase where they were shown a range of images and asked to determine which were genuine and which were fake. This training phase provided feedback, effectively improving the participants’ eye for spotting AI-generated images.
Once this phase was complete, the full study began. The participants were each shown 200 images, 50% of which were fake and 50% of which were real, and they had to choose which was which.

Layer 6 chose Prolific to source the participants it needed. A member of the research team recommended the partnership - they also read multiple studies showing that using Prolific for the tasks they were running would lead to better-quality data, which reaffirmed their decision.
Layer 6 worked with Prolific to source a sample of over 1,000 evaluators, specifying university-educated, English-speaking adults.
When asked what set Prolific apart from other competitors, like Amazon’s MTurk, it boiled down to a question of trust. Specifically, whether the team could be sure that selected participants were real people and not bots.
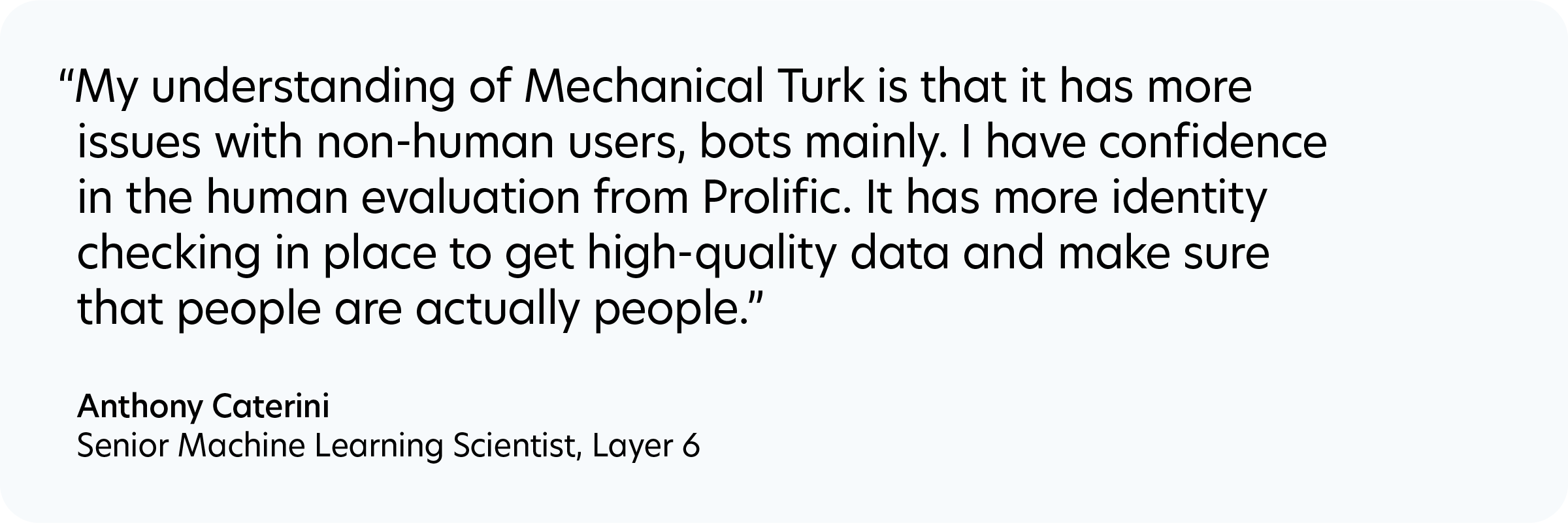
The results spoke for themselves - of over 1,000 participants, only two or three had to be removed for providing low-accuracy responses.

A member of the Layer 6 team had previous hands-on experience using Prolific in previous studies, so they knew that there was more to it than a robust data set. Thanks to responsive customer service and an API to run their own code before sharing with participants, they knew they were in good hands.
Jesse Cresswell, Layer 6 team member and collaborator on this study, was also impressed by the speed of data collection, noting that “running the study and recruiting people was extremely fast.”
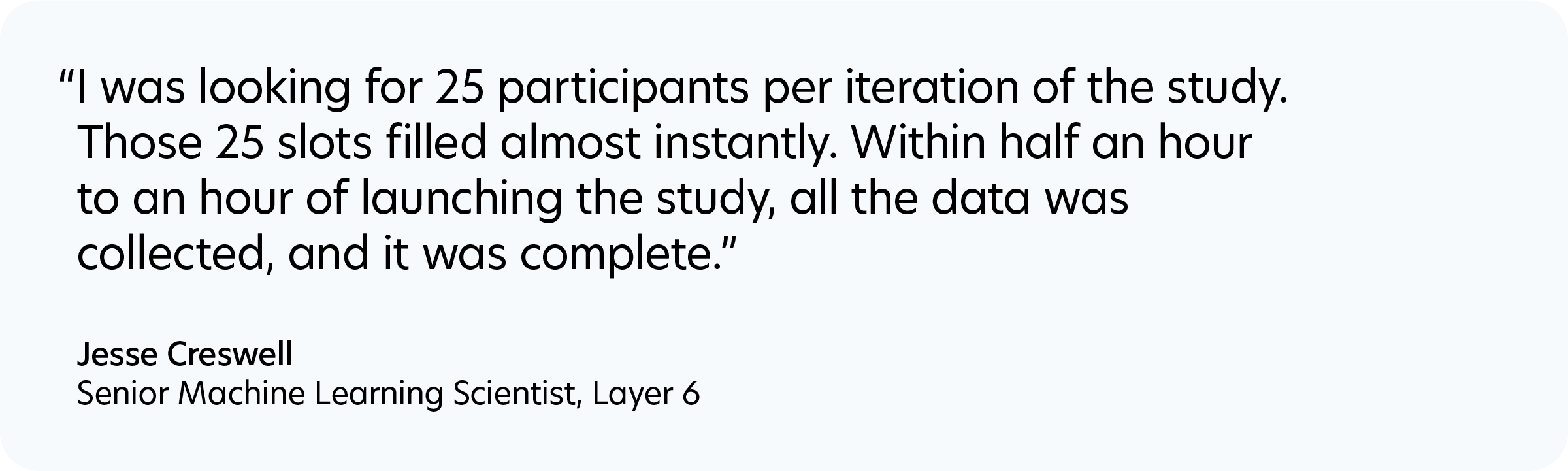
Key findings
In Caterini’s words, the team “found that the existing metrics do not correlate well with the human perception of quality of generated images,” confirming what they expected.
It turns out that the embedding model is key to these findings, as when it had a similar structure to GANs it was unfairly favoring GAN images over a diffusion model. In their search for a better option, they found the more advanced embedding model, DINOv2. Proving that it correlates better with human evaluation, they now recommend DINOv2 for use in future evaluations.
This groundbreaking research, published in 2023, is already gaining traction in the AI world. It has been published in the world's top academic machine learning conference, NeurIPS, and has been cited in follow-up studies by NVIDIA, Google, and Meta.
Real improvements, practical conclusions
What’s next for Layer 6? Caterini is now working on building tabular foundation models. He says that while “pretty much any science problem can be converted into a tabular data problem, foundation models for tabular data have lagged far behind, say, language models.”
Cresswell recently conducted a study published at ICML 2024 on how humans can benefit from AI suggestions when solving problems. He chose Prolific for the human data set, once again proving that Prolific is the top choice for Layer 6 AI.
If your AI research needs rapid evaluation from real people, Prolific can help. You can easily source samples of all sizes from our vetted pool of over 200k+ active respondents. Find out more.