How Shovels found high-skilled annotators for data labeling - with Prolific's specialist participants
Petra Kopić is a data engineer at Shovels, an intelligence platform for the building development and construction industry. Recently, she has been using Prolific to recruit specialist participants for an all-important task: labeling building permits to create a high-quality dataset for training and validating their AI models.
Prolific’s pool of specialist participants has been instrumental in enabling this research.
Let's explore how.
Tackling messy permit data with AI
Shovels uses AI to clean, classify, and connect building permits to addresses, properties, contractors, residents, and building owners. There was just one problem: permit data is notoriously messy.
"Almost every jurisdiction in the US has its own permitting system, with different schemas, different permitting requirements and so on. And there are over 20,000 jurisdictions in the United States," Petra explains.
To make sense of this jumble, Shovels needed to develop a system to tag permits based on the descriptions and details within each permit. It came up with 30 different tags, including:
- New construction
- Accessory dwelling units
- Solar panels
- Roofing
- Remodeling
- HVAC installations
Initially, this all seemed fairly straightforward. Yet, the messy, unclear permit descriptions created a challenge that required a specialized solution.
Watch the full video case study.
The challenge of finding specialist participants
Shovels is committed to producing high-quality data. It recognises the vast potential of AI but is also acutely aware of limitations like hallucination—when an AI system generates information or answers that appear plausible, but are actually false or nonsensical.
"To ensure that this doesn't happen, we agreed to set up a rigorous process to validate our tags before exposing large language learning generated data to our customers," Petra says.
That process involved having specialists label a sample dataset to create what Shovels calls a "golden dataset" for AI validation. Finding specialist participants proved tricky, however.
Finding suitable specialist participants was tricky, however. Permit data is not always straightforward to interpret, requiring expert knowledge to decipher and label. And Shovels found that the results from other annotation platforms were poor, most likely due to the fact that labelers were not tagged by their areas of expertise.
"We initially tried a different data annotation platform, but it didn't really work that well," Petra recalls. "The results were poor, and I think the majority of responses were bot-generated, and from low-quality bots actually."
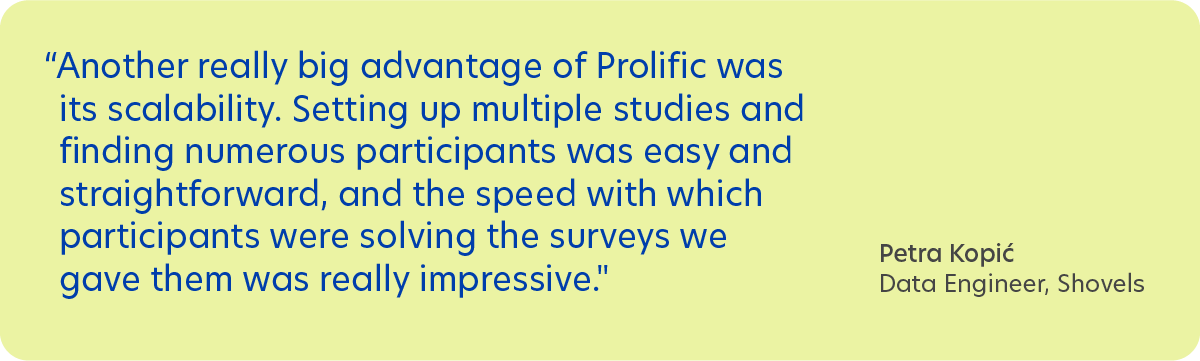
The solution for specialist participants: Prolific
Enter Prolific. Shovels used Prolific to find high-skilled, specialist participants to label building permits. These labels form the basis of quality datasets that become a baseline for the accuracy of their AI model.
"Prolific was a great choice," Petra says. "It made a significant difference for us because we easily found participants who were vetted and also experienced in the specific industry that we needed."
For Shovels, ‘specialist participants’ meant individuals in the construction industry with at least 100 similar questionnaires solved and an approval rate of more than 98%. It integrated Prolific with Argilla, an open-source platform for labeling and monitoring data for NLP projects.
"Another really big advantage of Prolific was its scalability," Petra notes. "Setting up multiple studies and finding numerous participants was easy and straightforward, and the speed with which participants were solving the surveys we gave them was really impressive."
This scalability was crucial. With 30 different tag categories, Shovels needed to guarantee their golden dataset included sufficient examples of each category—which required a large dataset.

Results that show iterative improvement and high data quality
Shovels' approach to measuring the accuracy of the labels was thorough. It had each permit labeled by multiple specialists, then analyzed the variances between their responses.
"Interestingly, in our initial study, we found significant discrepancies between their responses," Petra explains, taking this as a signal to refine their tags with experts in building, construction and real estate. "We went back, consulted with our advisors, and came up with a redefined set of tags. The following studies were much more successful, and we improved the consistency between the labelers."
This iterative process, supported by Prolific, allowed Shovels to refine their approach and improve their data quality.
The impact of enhancing product development and customer insights
Shovels’ high-quality labeled data, obtained by working with Prolific’s specialist participants, is having a real impact on product development and customer offerings, putting them in a position to effectively classify hundreds of millions of permits.
"LLM generated tags will really make a difference for us," Petra explains. "It will enable us to classify all of our permits into correct categories and enable our clients to find more insights."
For companies in the energy and sustainability sectors—some of Shovels' largest and most engaged customers—these insights are invaluable. They can use Shovels' data to identify homeowners likely to purchase building electrification equipment or to find qualified contractors for services like electrical panel upgrades and solar panel installations, to name a few.
"By looking at the building permit histories of homeowners, building owners, and contractors, Shovels can help climate tech companies target and match buyers with installers," Petra says.
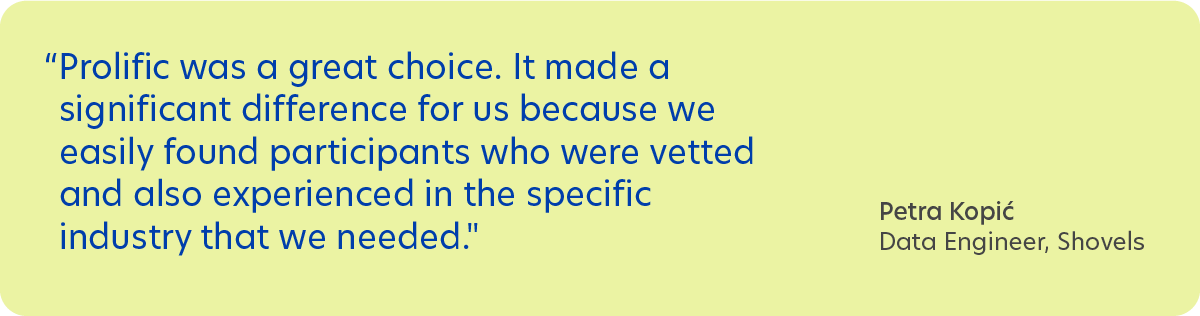
Looking ahead to future innovations and continued collaboration
Shovels' experience demonstrates the need for combining human expertise with AI capabilities. By using Prolific to access high-quality, specialist participants, it has created a robust dataset that serves as the foundation for accurate and reliable AI models.
As Shovels continues to innovate in the construction and climate tech industries, its partnership with Prolific will play a crucial role. "We really enjoyed this process, and we will use Prolific for our future validations," Petra concludes.
Summary: specialist participants for high-quality AI training data
For AI researchers and industry professionals facing similar data labeling challenges, Shovels' story offers a clear lesson: when it comes to creating high-quality training data, there's no substitute for human expertise. With platforms like Prolific, securing that expertise through specialist participants is more efficient than ever.
