Introducing Prolific's human-centered AI model leaderboard
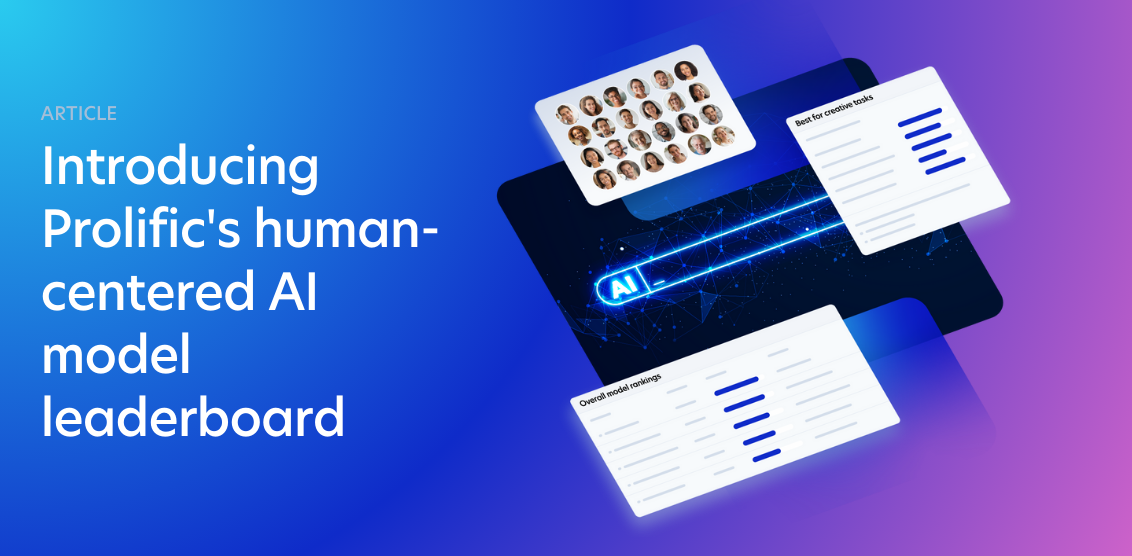
AI model leaderboards mostly focus on speed, tokens-per-second, and other technical metrics. But what about the human experience? How do people actually feel when working with these models?
That’s where Prolific's AI User Experience Leaderboard comes in. It's the first in our planned series of AI leaderboards that puts human feedback at the center of evaluating AI model performance.
Why we built a leaderboard
We believe quality human data is the key to building better AI. Traditional leaderboards provide valuable technical insights about AI models, measuring speed, reasoning abilities, and accuracy, among many other factors. These benchmarks are essential for advancing the field, but they tell only part of the story.
Our leaderboard represents our first step toward enhancing AI evaluation with the same principles that define our core work: rigorous participant verification and rich, detailed data collection.
The leaderboard adds new dimensions through:
- More detailed measurement beyond simple preferences
- A verified, representative participant pool and poststratification using MRP
- Controlled experimental conditions
- Rich, multi-dimensional feedback
It captures experiential metrics that matter to real users:
- Helpfulness
- Communication
- Understanding
- Adaptiveness
- Trustworthiness
- Personality
- Culture and representation
- Repeat use
Our approach shifts the focus. Instead of asking questions like "how fast can this model answer?" we ask "how satisfied are people with the results?"
Model proliferation has hit a new peak, with major releases from leading labs now a frequent occurrence. Claims about capabilities abound, but without reliable human feedback, it's hard to know which models truly deliver. Prolific’s leaderboard addresses this gap by providing a clear view of AI performance grounded in diverse, real-world user experiences - exactly what Prolific has always done best.
Limitations of existing benchmarks: Why a different approach is needed
Current AI evaluation methods face significant challenges that limit their usefulness for understanding real-world model performance:
Benchmark contamination undermines reliability
Large language models are increasingly trained on data that includes popular benchmarks designed to test them. Studies show contamination rates as high as 45.8% in some cases, with models effectively "seeing the answers" during training. This leads to artificially inflated scores that don't reflect true capabilities.
For example, portions of common datasets like LAMBADA and SQuAD have been found in the pre-training data of multiple leading models, rendering these benchmarks increasingly ineffective as true performance measures.
Technical focus misses user experience
Most current benchmarks prioritize abstract capabilities like mathematical reasoning, coding, or fact recall. While these skills are important, research shows they often fail to predict how satisfied users will be when using these models for everyday tasks. A model might excel at solving complex coding challenges but struggle with writing a helpful email or planning a simple trip—tasks that represent how most people actually use AI.
Non-representative evaluation creates blind spots
The gap between AI researchers and typical users is substantial. Studies show that when technical experts evaluate models, they tend to value different qualities than the general public and interact with models in ways that don't match typical usage patterns.
Even platforms like Chatbot Arena, which collect human preferences at scale, rely on unverified participants without demographic controls, completing an extremely diverse array of tasks that may not be directly comparable. This approach not only misses important insights about how these models perform across different user segments, but also the nuances underlying why a user may prefer one model over another as they only select high-level preference data.
Most benchmarks rely heavily on either expert evaluations or uncontrolled public feedback, missing insights about how models perform for their actual user base.
Limited real-world task alignment
Abstract puzzles and specialized tests dominate current benchmarks, but these rarely match how people use AI in practice. Research indicates that real-world AI usage centers around creative tasks, information seeking, planning, and decision support. These are the areas where technical benchmarks provide the least insight about performance.
These limitations explain why our leaderboard takes a fundamentally different approach to AI evaluation, one centered on real tasks, diverse participants, and multi-dimensional assessment of the user experience.
How the leaderboard works
We've built a leaderboard in partnership with ML Commons where participants directly engage with leading AI models to complete everyday tasks. Our participants work with the models on realistic tasks that reflect how people actually use AI in their daily lives.
Here's how it works:
- We use a representative sample of ~500 participants per release
- Each person completes six predefined tasks across six different models
- Models are presented in random order and fully anonymized
- After using each model, participants rate their experience across seven key dimensions: helpfulness, communication, understanding, adaptiveness, trustworthiness, personality, culture, and representation, as well as intention to use again
- Each dimension was also assessed through three specific sub-metrics (21 total), that attempted to determine why the overall score for that dimension was given, and providing deeper insights into participants' experiences
- We use a custom MRP pipeline to analyze responses to create both nationally representative overall rankings and detailed breakdowns by demographic groups
The tasks themselves cover common real-world scenarios: drafting emails, planning trips, explaining complex topics, making decisions between options, generating creative ideas, and developing meal plans.
What makes our approach different?
The Prolific leaderboard stands apart from others in several ways:
Real tasks, real people
Evaluation tasks are scenario-based and drawn from common naturalistic user journeys. Task prompts are balanced across cognitive and creative complexity, and models are evaluated in a blind, within-subject design to control for order and inter-subject effects.
Representative sampling
We use stratified sampling methods to recruit ~500 participants to make sure our results actually reflect how these models work for diverse users, as well as applying a further poststratification stage to weight our data by what we know about the US population.
Science-backed methodology
Our approach combines behavioral science principles with weighting methods used in public opinion research to limit potential sources of bias and create a highly representative, reliable, and generalizable dataset.
Demographic insights
All evaluations are segmented by demographic attributes to assess differential perception across user cohorts. It means model developers can identify audience-specific performance gaps and better align systems with target user groups.
Blind testing
Participants don't know which model they're using, which removes brand bias from their assessments and creates a more honest evaluation of the actual experience.
What's coming next?
The first leaderboard is now live, featuring head-to-head comparisons of six leading models: OpenAI o1, Llama 3.1 405B, Claude 3.7 Sonnet, GPT-4o, Gemini 2, and Deepseek R1.
We'll share insights about how different demographics perceive each model. The information will help AI developers understand how their models perform across various user groups and identify areas for improvement.
For AI model developers and research teams, our leaderboard provides valuable feedback on what real users care about. For researchers, it offers a fresh look at how we evaluate AI systems. And for everyone, it helps push the field toward building AI that really works for humans.
The data won't just live on a static page either. We’ve also built interactive elements that let you explore results by demographic factors, task types, and specific dimensions of performance. Want to know which model performs best for non-technical users trying to understand complex topics? Our filters show you exactly that.
We believe taking a human-centered approach to AI evaluation will help guide the industry toward building tools that better serve real needs. Our aim isn't to crown winners and losers, but to provide honest, useful feedback that helps improve these increasingly important technologies.
Discover the leaderboard
Evaluate your model
Are you developing AI models and want to understand how they perform with real users? We're expanding the models included in future leaderboard releases.
Contact us to discuss adding your model to our evaluation framework.
Join the initiative
The Prolific leaderboard is an evolving project that benefits from diverse perspectives. Whether you're interested in contributing to our methodology, partnering on research, or advising on future directions, we welcome collaboration. Reach out to learn how you can help shape the future of human-centered AI evaluation.