Potato and Prolific: Key takeaways from our study on bias in AI annotations
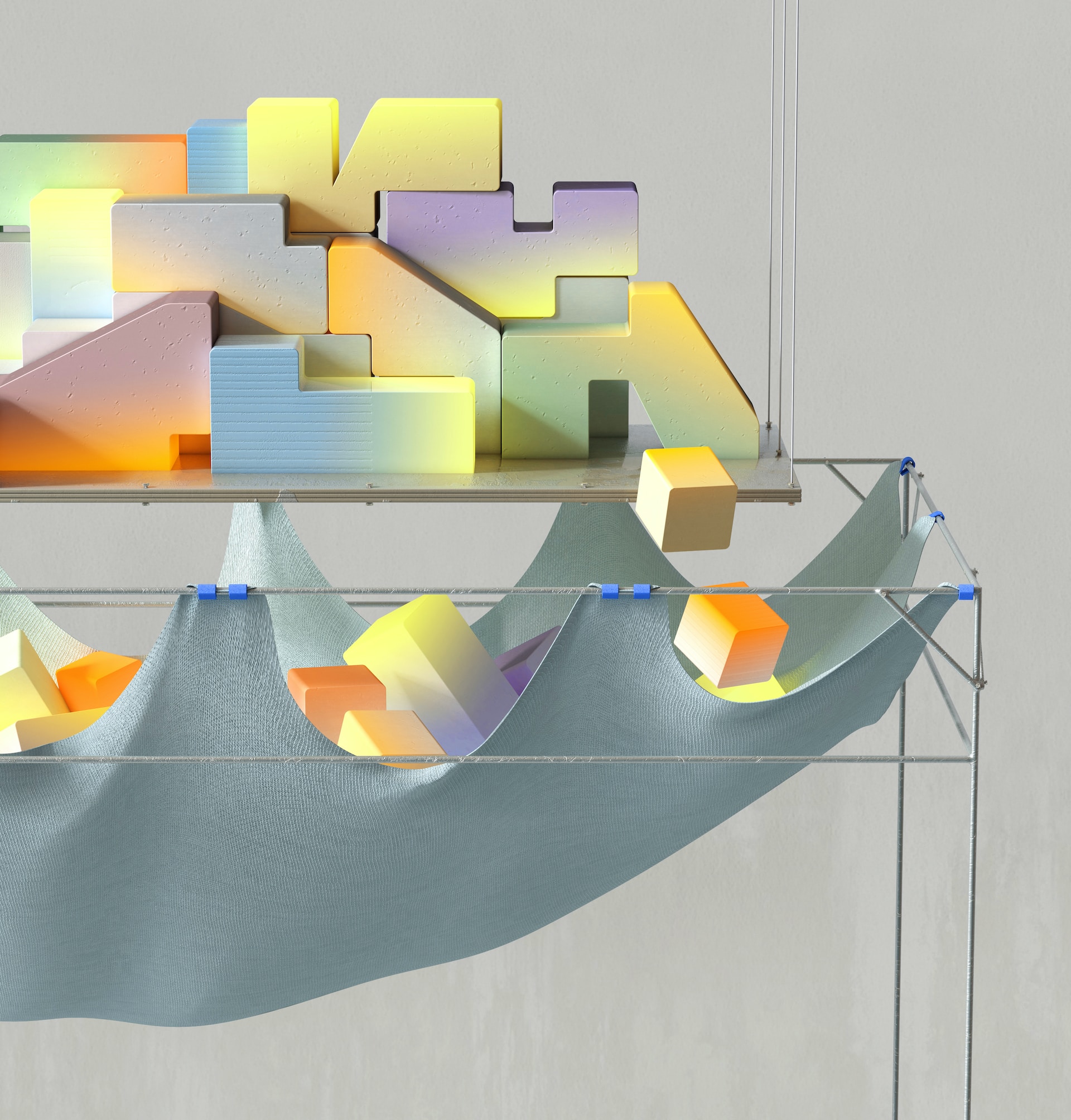
If you work in the AI space, you’ll know that the success of your models depends on the quality of your human annotation.
Typically, quality is measured through inter-annotator agreement (IAA). However, this assumes there’s a ‘ground truth’, or objectively correct answer, to any annotation - so any disagreements between annotators must be mistakes. While this may be true for some tasks, it’s not always the case.
With subjective topics comes an added layer of complexity: annotator biases. What one person finds offensive, another may find perfectly acceptable.
The question is, how much does annotator background matter when it comes to annotation tasks? Especially when reinforced learning with human feedback (RLHF) relies heavily on large-scale annotations from real, opinionated people?
The developers of Potato, a web-based solution for data annotation, ran an experiment with Prolific to find out.
About the study
Prior research on annotator background has mostly focused on specific aspects of identity, like gender, and on certain tasks, like toxic language detection.
We wanted to broaden this scope. So together, we picked four common natural language processing (NLP) tasks that asked participants to:
- Judge 1,500 comments from the Ruddit dataset for their level of offensiveness
- Answer question tasks from the SQuAD dataset by highlighting a response in a block of text
- Rewrite an email to make it more polite
- Rate the politeness of the original and new emails generated in the previous task
Thanks to our 130,000+ pool of diverse, engaged, and carefully vetted participants, we were able to split results by age, gender, and ethnicity. We used representative samples to ensure demographic splits. And we could run tasks with different degrees of difficulty, creativity, and subjectivity.
Here are some fascinating takeaways from the study you’ll want to read before recruiting your next batch of annotators.
Demographics matter. (A lot.)
In the task where we asked people to rate the offensiveness of comments, we found no significant differences in ratings between men and women.
But people older than 60 tended to perceive comments as being more offensive, compared to middle-aged participants. And Black participants tended to rate the same comments as being significantly more offensive compared to all other racial groups.
In our question-answering task, the largest differences in performance between demographics were seen with race and age variation, with a smaller effect for education.
These differences mirrored known disparities in education and economic opportunities for minorities compared with their White male peers in the US. The trend for age matches known results showing a moderate increase in reading ability with age.
And in our politeness-rating task, women judged messages as being less polite than men did. Older participants were more likely to give higher politeness ratings. And those with higher education levels tended to give lower ratings.
There were also significant racial differences. Black participants rated messages as being more polite than their White peers did. Asian participants gave the lowest politeness rating overall.
So, who annotates your data really matters. An annotator’s background influences their decisions, with varying degrees of subjectivity across different tasks – so it’s crucial to recruit with this in mind.
Also, ‘different’ doesn’t always mean ‘wrong’. In more subjective tasks, the varied decisions participants made weren’t mistakes. They were simply valid differences in views.
Bias breeds bias
Now, back to our offensiveness-rating task.
Interestingly, we found that scores by White annotators highly correlated with the original Ruddit dataset. Whereas those by Black and Asian annotators only moderately correlated.
This suggests the initial annotations are more likely to have been performed by White annotators – who may not have considered the offensiveness of certain comments for Black or Asian people.
It’s vital NLP papers that curate datasets consider whose voices appear in these. Because in the end, they decide whose thoughts are captured in models trained on the data.
Existing datasets might have been annotated by a group of participants with a demographic bias. This has a knock-on effect on any model using them to train.
The bottom line: biased data leads to more biased data – and so the cycle goes on, leading to objective models that can cause offence or provide shaky information.
What happened next?
After conducting our study, we:
- Released POPQUORN - This is a large-scale NLP dataset for four NLP tasks annotated by a representative sample of the US population with respect to sex, age, and race, which can be used by others to train their AI models.
- Used all our data to examine Prolific’s performance - Compared to existing annotations from curated workers, we were able to demonstrate that a general sample of Prolific participants can produce high-quality results with minimal filtering. This proves our platform is a reliable source of rich, diverse annotations.
If you’d like to try POPQUORN for yourself, or tap into our proven participant base for your AI research, head to our dedicated AI landing page and chat to our friendly sales team about your needs.
Or, read the paper in full – we’d hazard a guess it’s the most you’ll ever learn from a potato.