What is self-selection bias?
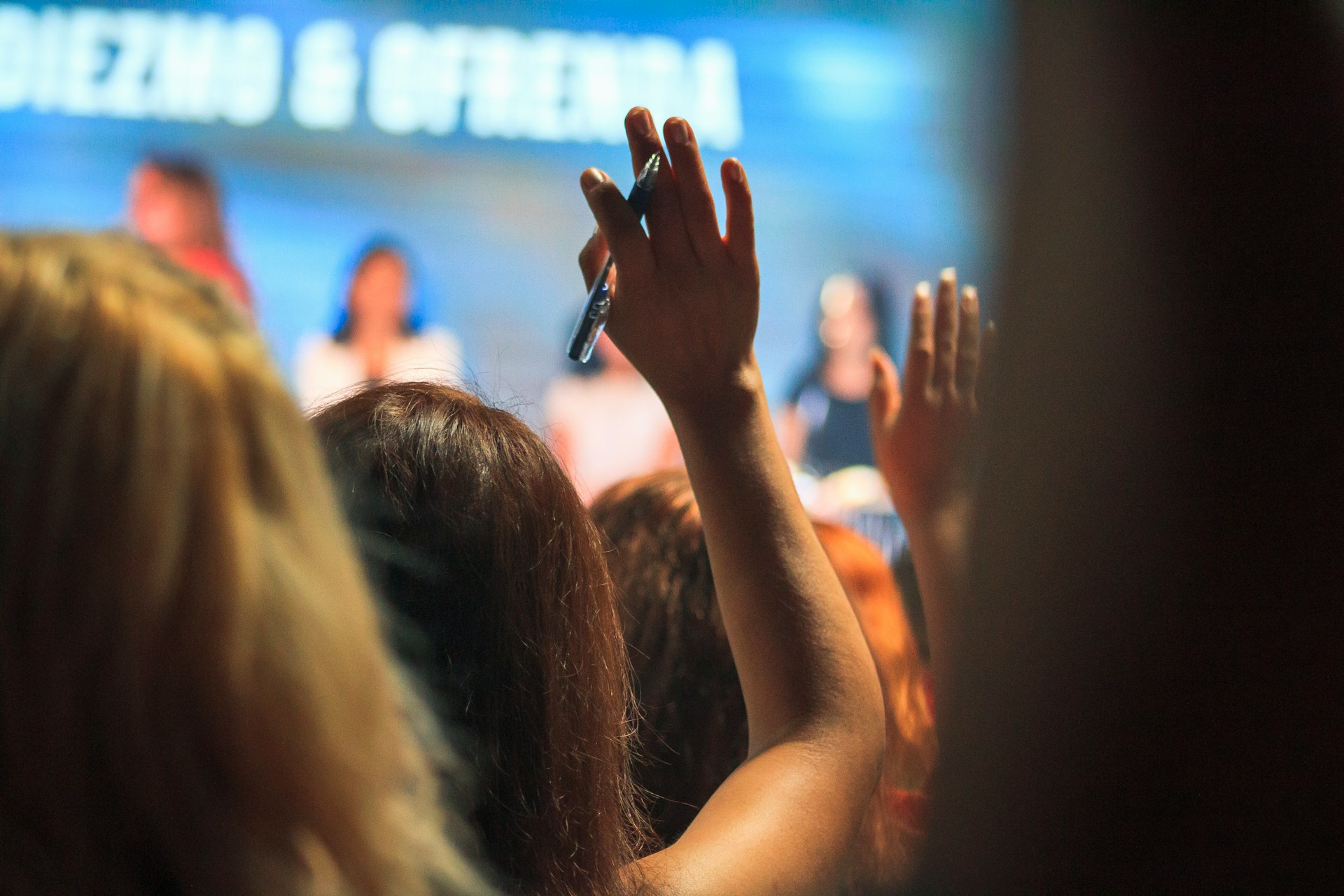
When you design a study, you’re setting out to address a research problem clearly, accurately, and with as little bias as possible. Good research design should eliminate bias at all costs. Otherwise, your findings risk being invalidated.
Assembling a group of participants who are representative of the population you’re studying as a whole is key. However, it can be all too easy to fall into various bias traps while doing so.
Imagine you’re putting out a call for people to take part in your study. You get a hundred positive responses and, after some due diligence with each, you take them all on board.
But this group of volunteers could be a skewed sample—and an example of self-selection bias.
What is self-selection bias?
Any situation in which people are allowed to put themselves forward carries the risk of self-selection bias. There’s a non-random link between all the participants—they want to take part.
The factors leading to their decisions will likely vary. But whatever they are, they can reduce your ability to extrapolate results from the answers that a group gives. They’re likely to have more free time than the rest of the population and more interest in the area of study. They might even be more intelligent and engaged than their peers.
All these factors can lead to inaccurate conclusions. This problem is magnified if the sample size is small or the bias goes unnoticed and uncorrected.
The impact of self-selection bias
There are several key problems that self-selection bias brings into play, each of which can place severe limitations on the usefulness of a study. These can include:
Voluntary response bias
Self-selected group members may have volunteered for reasons that could influence a study’s outcomes. This could be a pre-existing knowledge or interest in the subject of the study, their level of motivation or engagement with the department or institution, or a socio-economic background that supports the time to take part in studies.
An unplanned congruence could affect the results of the study in unpredictable and hard-to-detect ways.
Limited extrapolation value
A non-random group of respondents is less likely to accurately reflect the population being studied. This makes it challenging, potentially impossible, to make valid extrapolations from the data gathered.
An example of self-selection bias
Product updates are an area where companies have to tread carefully. A natural way of testing the potential impact of an update is to consult the existing user base.
For this example, let’s say a long-standing online game has been losing subscribers. The company that developed the game needs to arrest the decline.
To do this, they’re thinking about changing some core mechanics. To test the possible outcome, they sent out an invite to a survey, but only the most engaged players volunteered to take part.
As people who have invested significant amounts of their time and perhaps money in the company’s product, they’re potentially a valuable source of consumer-side information about that product. However, this view doesn’t take into account the often fervent views held by long-term users of a product as compared to the population as a whole.
Put simply, the more strongly the players identify with the game, the more likely they are to have deeply-held opinions on the game as they have known it. With this comes the high probability those views are much more polarized towards the extreme ends of the spectrum.
This leads to the strong possibility of self-selection bias. In this example, the company should recruit more widely, looking at more casual groups of players, and potentially also those who used to subscribe but have since canceled.
Tips for avoiding self-selection bias
There are a number of simple strategies that can significantly reduce the chances of self-selection bias becoming a factor. It’s not always possible to remove it completely but you can minimize its impact with some of the following measures.
Use random sampling techniques
First and foremost, use random sampling techniques when selecting participants. If they’re randomly selected rather than putting themselves forward, it will go a long way to mitigate self-selection bias.
Use screening questions in advance
You can use screening questions in advance to identify why potential participants want to join the study. This can help determine the degree to which their motivations might influence their answers and the study as a whole. If the degree is too great a risk to the validity of their answers, they can be removed from the pool.
Incorporate participant feedback
Build participant feedback into your process, both from those who chose to participate and those who declined. Both sets of information can help guide the analysis of your group.
Debrief regularly
Lastly, conduct regular debriefing sessions with your researchers and wider team to discuss the potential impact of self-selection, as well as other biases, on the study as it progresses. This can help pinpoint problems before they arise and should begin at the design stage.
Conclusions
Ultimately, recruiting highly engaged participants for your research is great for your data quality. While there is a risk of self-selection bias when people put themselves forward, you can minimize its impact by understanding its roots and through careful study design and practice.
To find out more about self-selection bias and how to avoid it, read our Complete guide to selection bias now.