How do data annotation jobs work?
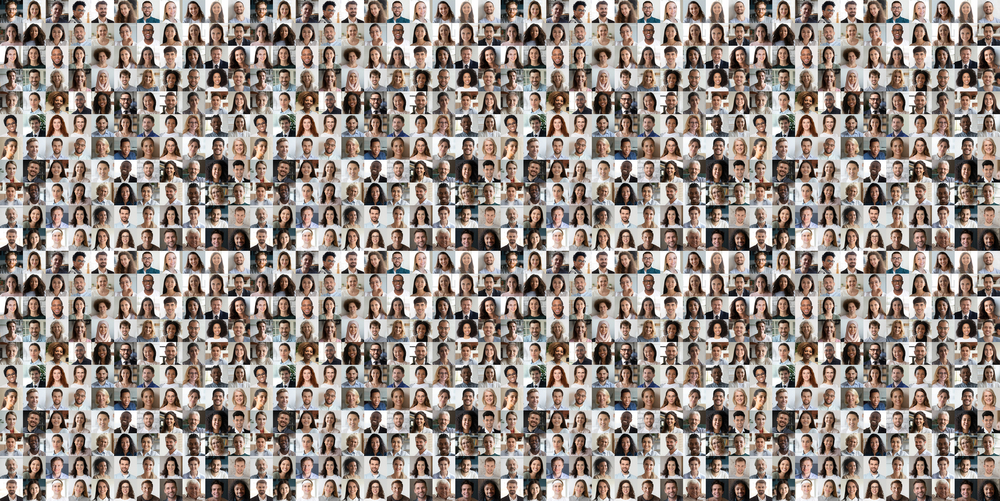
Data annotation tasks help create better AI systems by gathering human feedback on their performance. When you participate in these tasks, you'll review AI outputs and provide structured feedback that helps researchers understand what's working and what isn't. From quick ratings to detailed evaluations, there are many ways to contribute, and knowing how these tasks work makes it easier to get involved.
What happens in a data annotation task?
Data annotation tasks vary, but they all share some common elements. You'll receive something an AI system has created. It could be text, images, or even conversations. Your job is to evaluate specific aspects of this output based on clear guidelines.
A language task might ask you to rate whether an AI's response fits the question it was asked, while an image task could involve checking if an AI-generated picture matches its description. Some tasks need quick yes or no decisions; others ask for more detailed feedback about what works or needs improvement.
What makes these tasks valuable is their focused approach. Instead of general opinions, you're asked to look at specific elements of the AI's performance. This structured feedback helps researchers pinpoint exactly where their systems need work.
What are the different types of data annotation tasks?
Most data annotation work falls into clear categories. The tasks you'll encounter range from simple checks to complex evaluations, but each plays a specific role in improving AI systems.
- Text tasks, such as checking AI-written content for accuracy and natural language
- Image annotation like reviewing AI-generated images or helping AI understand photos
- Conversation evaluation includes testing how well AI chatbots maintain dialogue
- Safety testing is identifying potential issues in AI outputs
- Performance rating compares different AI responses to the same prompt
The complexity varies with each type. You might spend a few seconds deciding if an AI response answers a question, or take time working through a detailed conversation to test how well an AI system maintains context. What matters is providing clear, consistent feedback that helps researchers understand their systems' performance.
What makes for good annotation work?
The best data annotation combines attention to detail with good judgment. When you're reviewing AI outputs, focus on the specific aspects researchers have outlined while applying the same standards throughout your task.
Researchers rely on clear, honest feedback. If an AI response sounds unnatural, they need to know. If an AI-generated image has obvious flaws, that information helps them improve. The goal isn't to be critical or praise everything. You need to provide accurate assessments that highlight both strengths and weaknesses.
Be consistent
Apply the same standards to every item you evaluate. If you're rating conversation quality, use the same criteria throughout. Don't, for example, become stricter or more lenient as you go along.
Stay focused
Each task has specific elements you need to evaluate. Stick to what's being asked rather than getting sidetracked by other aspects of the AI's performance.
Provide clear feedback
When asked for explanations, be specific about what works or doesn't. Vague feedback like "this seems off" isn't as helpful as pointing out exactly where an AI response misses the mark.
Take your time
While some tasks need quick decisions, rushing leads to inconsistent results. Work at a steady pace that lets you give each item proper attention.
Watch for quality checks
Most tasks include measures to ensure reliable data. Pay attention to test questions and repeated items, as they help verify your feedback is consistent and accurate.
Where your feedback goes
Your data annotation work directly influences how AI systems develop. When researchers collect feedback from multiple participants, they look for patterns that show where their systems succeed or need improvement. Your ratings and comments might highlight consistent issues with how an AI handles certain types of questions, or show that recent changes have made responses more natural.
The feedback shapes the next round of AI development. Researchers use participant responses to adjust their systems, fine-tune how AI generates content, and verify when changes lead to better performance. They might focus on fixing specific issues participants identified, or explore new approaches when current methods aren't working well.
It’s an ongoing process. As AI systems improve, new rounds of testing help verify changes and identify fresh challenges. What starts as participant feedback often leads to meaningful improvements in how AI systems communicate, generate images, or handle complex tasks.
Getting started
Data annotation tasks need different skill levels. Some focus on general feedback anyone can provide. Other tasks might need specific knowledge or experience to properly evaluate technical or specialized content.
If you're interested in contributing to AI development through annotation tasks, Prolific connects participants with researchers running these types of studies. Creating an account lets you browse available tasks and choose ones that match your interests and expertise. Each task comes with clear instructions and examples, so you'll know exactly what researchers are looking for.