How to improve data quality in online studies
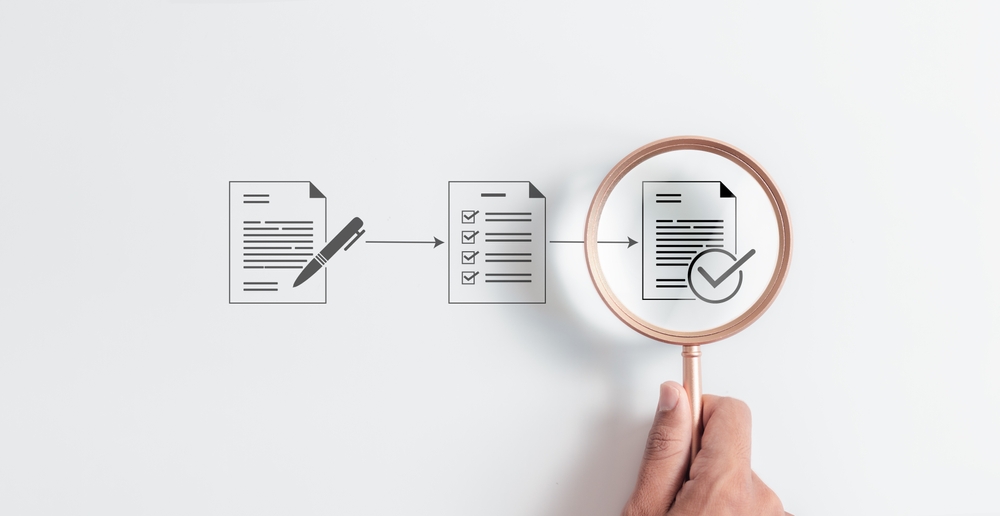
Online research has changed how we gather information. We can reach higher volumes of people, faster and more cost-effectively than ever before. An expansion like this, however, brings a significant challenge: how do we make sure the quality of the data collected is any good?
It's a concern for researchers. After all, what value does a study hold if its results can't be trusted? Recent studies suggest that up to 46% of opt-in panel respondents might be unreliable (Harmon Research and Grey Matter Research, 2020; PEW Research, 2019). The saying "Garbage In, Garbage Out" rings especially true here.
With these challenges in mind, we look at the steps to enhance your data quality and instill greater confidence in your findings.
Selecting the right platform
When it comes to online research, there are a number of platforms available to help facilitate the researcher’s needs. But they’re not all created equally. Some options consistently produce high-quality data, while others may leave you with more questions than answers. When evaluating the best platforms for your needs, consider the following criteria.
Does it have:
- Strong participant verification? (how the platform ensures that the participants that join it are real people).
- Continuous quality control of the participant pool? (Once the participant is on the platform and taking studies, what does the platform do to make sure they provide you with good data?)
- A large, active participant base? (Don't believe the marketing, as most participants in online pools are inactive and small samples have issues of naivety.)
- Features aligned with your specific research requirements? ( The tool should allow you to run the type of research you actually want to run)
Recent comparative studies have assessed major platforms based on key quality metrics. A study by Peer et al. (2022) found that Prolific outperformed other platforms on most measures, with MTurk behind in this area.
Participant engagement is equally important. Douglas et al. (2023) investigated this aspect, finding that Prolific offered the lowest cost per quality respondent compared to major providers (Qualtrics, Sona, Mturk, Cloudresearch).
It's also important to recognise that different software is suited to different needs. Some tools, like Qualtrics, are designed for running surveys, while others, such as Gorilla or Pavlovia, are tailored for experiments.
Again, Prolific shines here, connecting to hundreds of services and offering access to a wide variety of participants. As a result, Prolific makes it easier to fulfill the distinct needs of both surveys and experiments, offering easy access to diverse participant pools.
Trust, but verify
Once you've chosen your platform, the work isn't over. Take a proactive approach and verify that participants are who they claim to be.
Incorporate checks into your study design. Ask screening questions again to confirm accuracy, and keep demographic requirements confidential in the task description. Taking a subtle approach like this can help remove those attempting to manipulate the system.
Open-ended questions are invaluable in this process. They require participants to engage more deeply and provide insight into their thought processes. You should also be alert for inconsistencies in responses. For instance, if a participant claims to be a lifelong vegetarian but later mentions their favorite steak recipe, it may indicate unreliable data.
Consider verification as a method to uphold the integrity of your research, rather than a sign of mistrust. Implementing these checks means you're improving the quality of data while also showing respect for the participants who are genuinely committed to contributing to your study.
Designing for quality
A well-constructed study is your best defense against questionable data. Focus on getting the fundamentals right so everything else falls into place.
Include free-text responses
Always include at least one free-text response, though more is preferable. These are harder for low quality participants to bypass easily. Additionally, include duplicate questions with multiple options. Attentive participants should provide consistent answers across these questions.
Free-text responses not only help validate participant engagement but also allow you to catch potential automated or AI-generated answers. They're helpful for assessing the quality and depth of the thought participants are putting into your study.
As Veselovsky and colleagues (2023) point out, analyzing these responses can help you spot signs of large language model use, which is an emerging concern in online research.
Set appropriate filters
Ex-ante filters are pre-set criteria used to select participants for a study before it begins. When setting them, aim for a high approval rate—on Prolific, you can set this higher than 97% since we already remove most participants below this threshold. Look for participants with a history of completing 20 or more studies. If language fluency is necessary for your research, make sure to include this in your filtering criteria.
These filters, as highlighted by Tomczak et al. (2023), can significantly improve data quality. They help with recruiting participants who have a track record of providing reliable responses. However, be mindful that overly strict filters might limit your participant pool or introduce unintended biases.
Strike a balance between quality and inclusivity to get the best results for your specific research needs.
Monitor response timing
Collect data on the time participants spend on each page. Doing so helps identify those who may be multitasking during the study or not giving it their full attention. Don't rely too heavily on overall completion time. Individual page timing data gives you a much more accurate picture of participant engagement. The goal is to have dedicated participants, not those dividing their attention.
As Ramsey et al. (2023) point out, page timing data is a necessary tool for assessing response quality. It can help you spot both those who rush through your study and ones who take suspiciously long, possibly indicating distraction or consultation of external sources. When analyzing this data, look for patterns that align with the cognitive demands of your questions.
Remember that extremely fast or slow responses aren't always invalid, but they do warrant closer examination.
The art of attention checks
Attention checks are necessary checkpoints designed to see if participants are paying attention and properly engaging with the questions in your study.
Never rely on a single attention check. Even the most effective check isn't a perfect measure. Incorporate several throughout your study, but be cautious not to overdo it. Doing so can irritate participants and potentially reduce the quality of their input.
Keep your checks relevant to your study content. The goal is to assess current attention, not test prior knowledge or memory. Simple instructional manipulation checks like "What color is a red apple? Please select 'green' to show you're paying attention" aren't very effective, with research showing that participants can often pass these checks even when performing poorly on other quality measures.
Instead, use more reliable attention checks like non-sensical questions or bogus items, which better measure genuine participant attention. These methods are proven to be more reliable indicators of data quality and integrate naturally into your study flow.
Data analysis and quality control
Once your study is complete and responses are in, you’ll need to distinguish the valuable insights from the noise.
- Start by examining completion times. Unusually quick completion might indicate participants aren't reading thoroughly. Don't just look at total time—analyze time spent on individual pages. Using page timing data can reveal important patterns about how participants are interacting with your study.
- Again, free-text responses are invaluable for quality checking. Be wary of short, vague answers, and look out for copied text or responses that don't align with the question.
- The age of AI also brings its own challenges: spotting machine-generated responses. Keep an eye out for unnaturally perfect grammar or responses that sound a bit too generic. There are tools out there to help with this, but they’re not always accurate. A keen eye is still your best defense.
- Don't overlook the basics. Check for duplicate IP addresses, look for straight-lining (where participants select the same response option repeatedly), patterns of random clicking, and signs of low-effort responding.
- While time-consuming, taking an investigative approach is necessary for guaranteeing the quality of your final dataset.
With these processes, you’ll focus on maintaining the integrity of your research while valuing the time and effort of your genuine participants.
Treating participants as individuals
Your participants aren't just data points; they’re real people. Treat them well, and they'll return the favor with high-quality responses.
One way to do this is by paying a fair rate. It's not just about ethics (though that's important too). Studies (Largent et al., 2022) show that fair pay boosts recruitment and retention. You get a larger pool of people who are more likely to engage fully with your study.
Keep your study engaging by varying stimuli and question types. A monotonous series of similar questions can lead to disengagement. Variety helps maintain participant interest and encourages thoughtful responses.
Approach your research with a positive mindset. Most participants genuinely want to contribute to your research's success and aren't intentionally providing poor data. Create an environment where participants feel valued and respected, and you'll likely see this reflected in the quality of their responses.
Practical application: A case study
Consider a public health researcher investigating the impact of social media on mental health across different age groups. They plan to survey 2,000 people in the US, ranging from teenagers to older adults.
After careful platform selection based on data quality metrics, they set up pre-screening criteria for US residents aged 18 and above, stratified into age groups. These criteria are kept confidential in the study description to prevent self-selection bias.
The study design combines various question types, including multiple-choice, Likert scales, and open-ended responses about social media habits and self-reported mental health indicators. Three subtle attention checks included in the survey, and question blocks are randomized to mitigate order effects. The researcher also implements page timing to track engagement levels throughout the study.
Following ethical payment guidelines, the researcher offers $12 per hour, guaranteeing fair compensation for participants' time and effort.
During data analysis, the researcher flags responses completed unusually quickly (more than one standard deviation below the mean completion time) for closer inspection. They use text analysis tools to identify potential AI-generated responses in the open-ended questions. Additionally, they cross-check responses to similar questions placed at different points in the survey to ensure consistency.
The resulting dataset provides a comprehensive, high-quality representation of social media's impact on mental health across US age groups. The researcher can confidently submit their findings to peer-reviewed journals and present recommendations to health policymakers, knowing their data can withstand rigorous scrutiny.
Future trends in online research
The world of online research is always evolving. New challenges arise, but so do new solutions. Here's what to keep an eye on:
Advancements in AI detection
As AI-generated responses become more sophisticated, researchers must stay ahead of the curve. While new tools are constantly emerging to address the issue, the most effective techniques still involve careful scrutiny of responses to identify anything that sounds unnaturally perfect or generic. Some platforms now include built-in measures to detect this kind of unusual, AI-like behavior.
Increasing focus on participant quality tracking
Platforms are developing more reliable historical quality metrics. Some researchers are even creating their own databases of high-quality participants for longitudinal studies.
High-quality data
Obtaining high-quality data in online research shouldn't be left to chance. It requires careful planning, execution, and analysis. By following these steps, you can enhance the reliability and validity of your research:
- Choose your platform wisely, prioritizing quality.
- Verify your participants while maintaining trust.
- Design your study with built-in quality checks.
- Conduct thorough data analysis and cleaning.
- Treat participants fairly to encourage engagement.
Online research is powerful. With the right approach, you can harness that power to uncover insights that really make a difference. Armed with these strategies, you're well-equipped to tackle a wide range of research questions and contribute meaningfully to your field of study.
The goal isn't just to collect data, but to gather insights that can withstand scrutiny and drive informed decision-making. By prioritizing data quality at every stage of your research process, you're contributing to the advancement of knowledge in a responsible and impactful way as much as you’re simply conducting a study.
Enhancing your research with Prolific
Prolific is a leading online platform designed by researchers, for researchers. With over 200,000 active, vetted participants and a commitment to high-quality data, Prolific can help you elevate the quality of your online research:
- Access a diverse, engaged participant pool verified through bank-grade ID checks.
- Use 300+ demographic filters to find your ideal participants.
- Benefit from continuous quality monitoring with 25 different algorithmic checks.
- Design flexible studies using text, imagery, voice, or interaction.
- Scale your research efficiently with API integration.
Experience the difference high-quality data can make in your research with Prolific