What are AI ethics? 5 principles explained
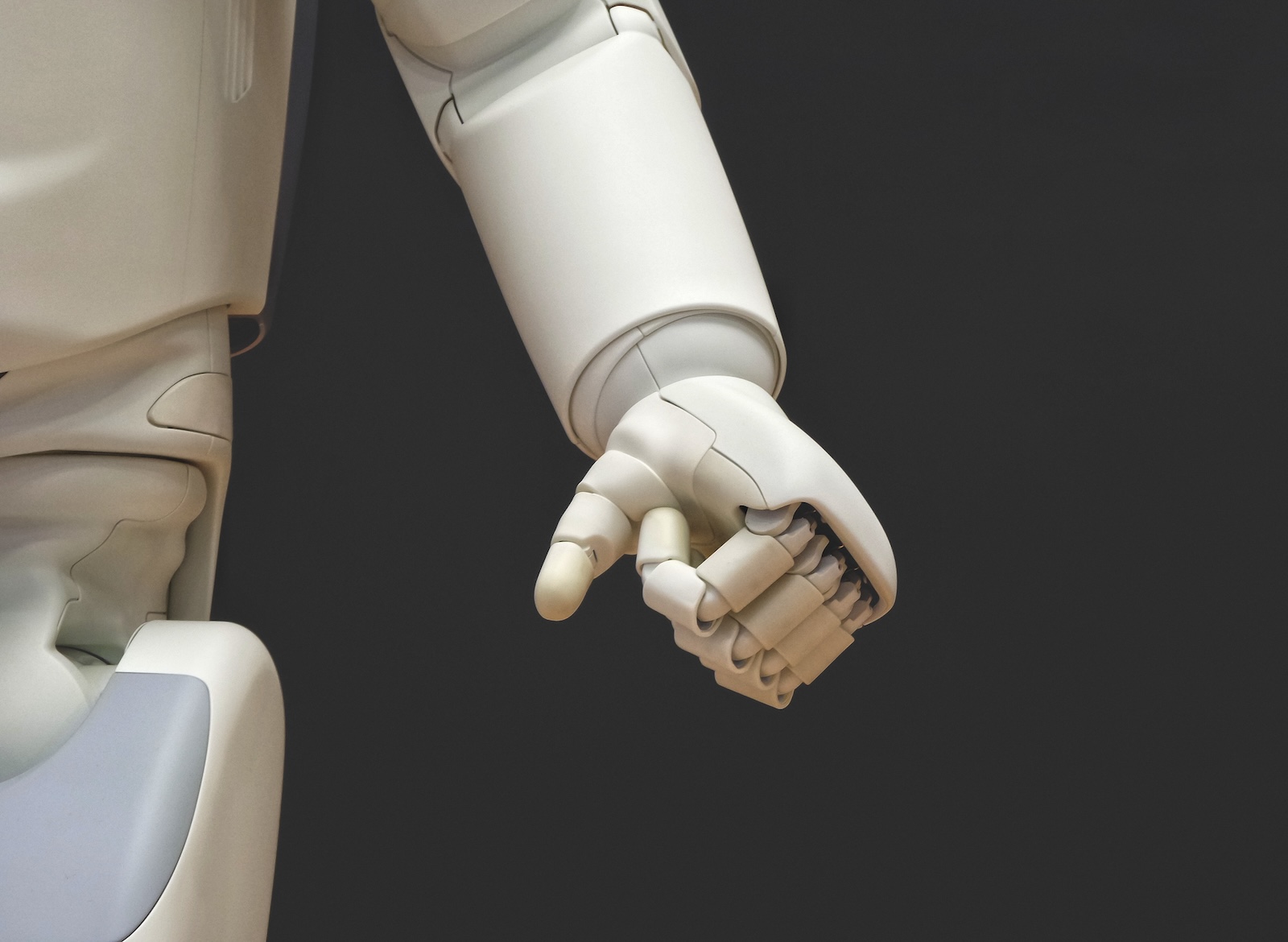
People have been talking about AI ethics for over 70 years.
Isaac Asimov first introduced the famous three laws of robotics in his 1942 short story, “Runaround”. Back then, AI ethics were science fiction. Today, it’s a very real concern for every AI researcher. According to the Capgemini Research Institute, AI creates ethical issues in at least 9 out of 10 businesses.
But what are AI ethics?
In this blog post, we’ll take you through the key principles of AI ethics, explaining what each principle means, why it’s important, and how high-quality data is pivotal to ethical AI.
What are AI ethics?
As AI technology becomes more advanced, ethical issues are more likely to arise. Artificial intelligence simulates human intelligence and decision-making. Unfortunately, this comes with many risks, including those to human safety.
AI needs a lot of data to work. If that data is inaccurate or biased, it can lead to poor-quality or even dangerous output.
AI ethics are a set of principles and guidelines for how we develop and use AI. Organizations incorporate these formally into AI ethics policies. This ensures that decisions made by staff and stakeholders are kept within ethical AI guidelines, minimize risks, and focus on improving life for all human beings.
5 key principles of AI ethics
1. Transparency
From hiring processes to driverless cars, AI is integral to human safety and wellbeing. That's why AI systems need to be transparent. Businesses, customers, and the wider public need to understand how the algorithms work and why AI has made certain decisions.
For example, a bank might refuse a customer an online loan. The customer will naturally want to understand why the algorithm refused their application. With this information, they can potentially improve their chances of approval in the future.
The Dutch government is preparing to introduce a register that will require public services across The Netherlands to publish their AI algorithms online. However, some have argued that this is the wrong way to improve transparency. Why? Most people won’t be able to understand the data. For an AI system to be transparent, developers need to be clear about how their AI makes its decisions. Meanwhile, public understanding of artificial intelligence also needs to be improved.
2. Impartiality
Another key principle for AI ethics is impartiality. AI should treat all human beings equally. That means eliminating bias and discrimination from AI systems. How can you achieve this? With high-quality data. Many data sets are not specifically created for training AI. When they’re used for this purpose, they can pass on quirks and biases from the data collection process.
Artificial intelligence can’t pick up on biases within its data. If this isn’t addressed, AI systems could repeat these biases and implement them automatically. There have been many cases of AI bias upholding systemic forms of discrimination towards marginalized groups. That’s why researchers must use unbiased, high-quality data and test models to see if they display biased behavior.
3. Accountability
Accountability is another important aspect of AI ethics. Algorithms are run by artificial intelligence. So, who is held accountable when something goes wrong? People and organizations who have worked on an AI system need to be held accountable at each stage of the process, not just after the AI is already operating.
With AI accountability, prevention is as important as the cure. Teams need to ensure they understand how well the system is working, supervise the development of the algorithms, and select high-quality data to feed into the system. Organizations should consult diversity experts, as well as people who will be using the AI system. What’s more, if an AI system is used for sensitive purposes, such as public services, it should always be held accountable by external review.
4. Reliability
AI systems need to be reliable. This ensures that the results achieved by the system are reproducible and consistent. That’s especially important when AI is being used for an important service, such as healthcare or credit applications.
Monitoring AI systems is key to ensuring their reliability. This way, any issues are immediately reported, and measures can be put in place to mitigate risks.
5. Security and privacy
Security measures need to be established to ensure that sensitive data is stored and used securely. These measures include data encryption, locating system vulnerabilities and defending against malicious attacks. Responsible data collection and robust data governance practices are also essential.
According to Forbes, one of the biggest challenges is that AI is often patched together by a network of very different creators. This makes it hard to achieve the levels of accountability, reliability, and security needed for ethical AI. To become truly secure, you need a unified approach to security across the entire lifespan of the AI system.
Ethical AI hinges on high-quality data
So, what are AI ethics in practice? Ultimately, it’s all about data! That includes everything from the way you use it to the quality of the data you collect. Poor-quality, biased data leads to poor outcomes. As we’ve shown, AI bias has a hugely detrimental impact on businesses, researchers, and the wider population.
Find out more about creating ethical AI in The Quick Guide to AI Ethics for Researchers. You'll discover 6 key ethical challenges you need to be aware of, plus 4 essential tips to help you train AI ethically and responsibly. Download your copy now.