What are the different types of data annotation jobs?
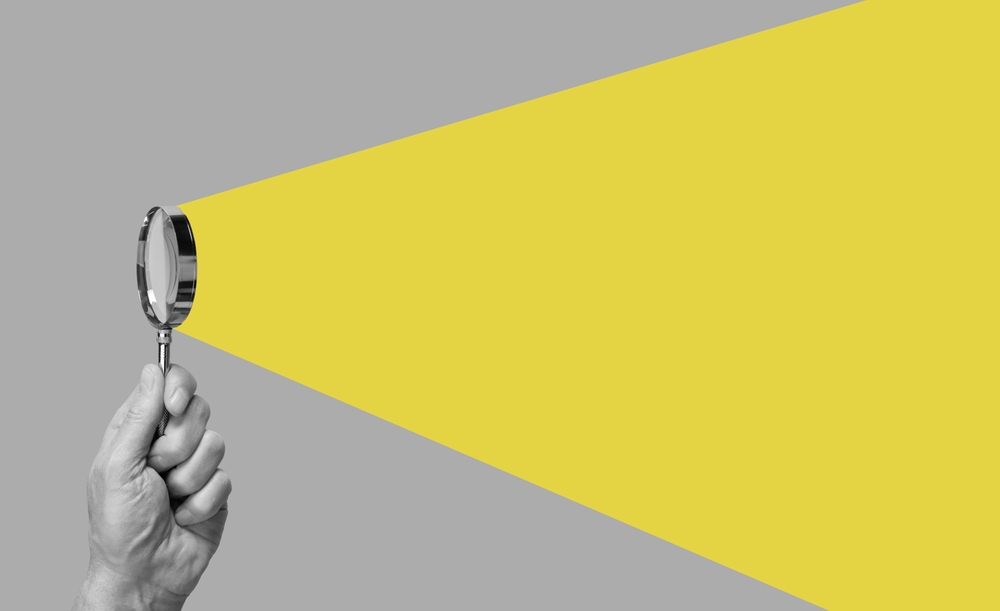
AI development relies heavily on human input, and researchers on Prolific regularly seek participants for various annotation tasks. Whether you're rating language outputs, testing interactions, or providing feedback on AI tools, each task type brings its own rewards and challenges. We look at the different types of annotation work you might encounter as a research participant.
Text annotation and evaluation
Text-based tasks make up a significant portion of AI research studies. Many focus on making AI communication more natural and helpful by gathering human feedback. You might evaluate how well a chatbot responds to questions, checking both the accuracy of its information and whether it communicates naturally. Researchers often ask participants to compare different AI responses to the same prompt, helping them understand which approaches work better.
Some studies involve reviewing longer content, like checking if an AI-written summary captures the key points of an article or document. These tasks require reading and thoughtful feedback about what the AI system did well or missed. Your insights help researchers refine their systems to better understand and communicate information.
Image annotation tasks
Participants help AI systems improve their understanding of visual content when working with images. These tasks vary widely, from basic object identification to evaluating complex AI-generated artwork. For instance, you might review whether an AI system correctly generated an image based on a text description, or compare multiple AI-generated versions of the same concept.
One aspect of image annotation involves helping AI systems understand context and appropriateness. With the right feedback, AI image generation becomes more accurate and reliable while avoiding problematic content.
Conversation tasks
Many studies need participants to engage in extended dialogue with AI systems. Unlike simpler evaluation tasks, these conversations require back and forth interactions with the AI, often across multiple exchanges. This helps researchers understand how their systems handle natural conversation flow and maintain consistent, appropriate responses over time.
Conversation tasks may include:
- Testing natural dialogue flow with AI chatbots
- Evaluating how well AI systems maintain context
- Rating appropriateness of responses
- Checking for consistency across conversations
- Providing feedback on tone and personality
Conversation tasks can be particularly engaging since they often mirror real-world interactions. You might test how well an AI system remembers details from earlier in the conversation or whether it maintains a consistent personality, or if it can adapt its tone appropriately to different situations.
Safety and content evaluation
AI systems should operate safely and appropriately so there aren’t issues around misinformation or bias. Participants often review how systems handle various scenarios and identify potential issues. The work goes beyond simple content checking, and you might evaluate whether an AI system recognizes when it should decline certain requests or how it handles sensitive topics.
This feedback also helps researchers understand how their systems perform in real-world situations and where they need additional safeguards. A careful evaluation process helps develop more responsible AI systems that better serve users while avoiding potential harms.
Specialized tasks
Some researchers seek participants with specific expertise or knowledge areas for specialized projects that go beyond general feedback. They often require informed perspectives on technical or professional content to properly assess AI performance for things like:
- Technical documentation review
- Scientific content assessment
- Educational material evaluation
- Creative writing analysis
- Code and programming feedback
Having a background in these areas proves particularly valuable to researchers. A programmer might spot subtle issues in AI-generated code that others miss, while someone with teaching experience brings insight to educational content evaluation. With specialized feedback, researchers can develop AI systems that can handle complex, field-specific tasks with greater accuracy.
Making the most of your participation
Success in data annotation tasks comes from understanding what researchers need and providing thoughtful, detailed feedback. Take time to read task instructions, as requirements can vary between studies. While some tasks need quick, decisive responses, others benefit from a more detailed explanation of your reasoning.
When choosing tasks, consider both your interests and expertise. If you have specialized knowledge in areas like programming or education, look for studies that can benefit from your background. For general tasks, your everyday experience as a technology user provides valuable perspective on how AI systems should interact with people.
Most participants find they develop a better eye for evaluation over time. You'll start noticing subtle differences in AI responses and understanding what makes some interactions more successful than others. As a result, your feedback will be increasingly valuable to researchers.
Shaping what comes next for AI
Participation in these data annotation tasks directly influences how the systems develop. Whether you're helping improve everyday chatbot interactions or contributing to specialized research projects, your feedback shapes how these technologies will work in the future. Researchers value this real-world perspective, as it helps them create AI systems that better serve actual users.
Ready to contribute to AI development? Create an account on Prolific to browse available studies. You'll find a variety of tasks, with researchers posting new projects regularly.