How to avoid sampling errors in your research (with examples)
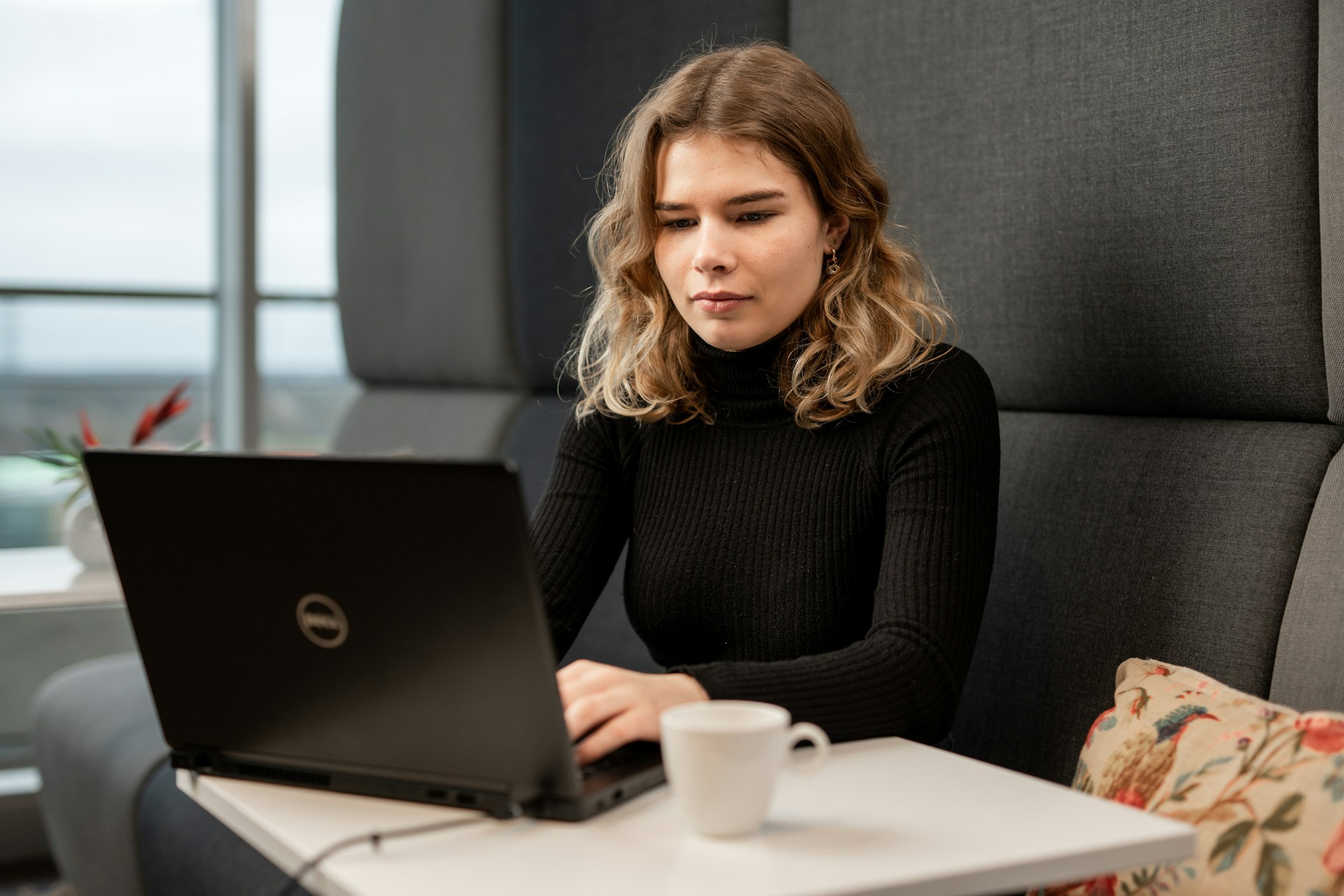
Trusting the results of your research might sound simple. However, despite thorough preparation, some errors and biases may creep in—like sampling errors.
Sometimes, the entire population you want to study can’t be included in your research, so you select a sample group to survey. Sampling errors happen when the sample you collect is unrepresentative of the target population or exhibits undesired bias or skew. Fall victim to an error large enough and you may have to start your study all over again, wasting valuable time and resources.
But sampling errors don’t have to be fatal. With the right procedures, you can reduce the effects of sampling errors on your results.
What is a sampling error?
Before we understand sampling errors, we should understand sampling itself. Sampling is the act of selecting a small subset of the population you’re studying. That way, you gain insight into a population without having to include every subject within that population.
It’s a bit like when swimming pools test the water. They take a vial of water to assess instead of testing the whole pool. In terms of participant-led studies, sampling involves selecting a smaller group of people to survey, who represent the wider population being studied.
A sampling error is the difference between a sample statistic and the actual population parameter it aims to estimate. This discrepancy occurs naturally due to random variation in the sampling process, even when proper sampling techniques are used.
The presence of sampling error means that sample results may not perfectly reflect the overall population characteristics. Consequently, findings based on the sample may deviate to some degree from what would be observed if the entire population were studied. This deviation doesn't necessarily make the results invalid, but it does introduce a level of uncertainty that researchers must account for.
To minimize sampling errors, researchers can employ several strategies:
- Increase sample size, as larger samples tend to more closely represent the population.
- Use proper random selection methods so each member of the population has an equal chance of being included in the sample.
- Employ stratified sampling techniques to showcase representation of important subgroups within the population.
- Use statistical methods like confidence intervals to quantify and report the potential range of sampling error.
It's important to distinguish sampling errors from non-sampling errors, which are caused by factors in data collection unrelated to sample selection, such as measurement errors or respondent bias. Sampling errors are also different from sampling bias, where systematic factors cause the sample to be unrepresentative of the population from the outset.
Types of sampling errors
Sampling errors typically fall into one of four types. Breaking down the error to its cause helps you understand how to improve your research method and decide which approach to use going forward.
Population-specific
These errors occur when multiple populations are potential subjects for the study. This situation arises when different groups share relevant characteristics or behaviors that align with the research objectives. For example, if you're studying consumer habits, both younger and older demographics might be relevant. Deciding which population to focus on is necessary, as studying the wrong group could lead to results that don't align with your research goals.
Selection
Selection errors happen when the sample doesn’t accurately represent the population because certain groups are over- or underrepresented. This can occur if the sampling method unintentionally excludes or favors particular demographics.
For example, a clothing brand conducting market research may inadvertently focus on a demographic that is easier to reach, missing out on the views of potential customers. Using random sampling and broader outreach strategies can help reduce selection errors.
Sample frame
Sample frame errors occur when the list or source used to select the sample (known as the sampling frame) is inaccurate or incomplete. This means the sample drawn doesn't represent the intended population. To reduce sample frame errors, make sure the sampling frame is accurate and up-to-date, reflecting the current population as closely as possible.
Non-response
If you have people who don't respond to the survey, then it may be that those people are from a specific group and their non-response creates a resultant bias or skew in your sample.
Examples of sampling errors
Let’s look at things more practically with these examples of sampling errors.
Example 1
A mobile phone company wants to survey customer satisfaction for a new smartphone model. They send out surveys to current users of their older models but exclude non-users.
This leads to a selection error, as the sample only represents existing customers who may already be loyal to the brand. The survey may fail to capture the opinions of potential new buyers or those who switched to competitors, providing a skewed view of overall satisfaction.
Example 2
A university conducts a survey to evaluate student satisfaction with its online learning platform. The survey is emailed to students who actively logged into the platform within the last month.
This introduces a non-response error, as it excludes students who haven't logged in recently, possibly due to dissatisfaction with the platform. By missing out on this group, the survey may overestimate satisfaction levels and fail to accurately represent the views of the entire student body.
How to eliminate sampling errors
Fortunately, there are ways to minimize sampling errors in your study.
Study the population
Research the population before you start. Then, measure the demographics and incorporate them into your sample data set. For instance, if 45% of the population identify as women, make sure that 45% of your sample identify as women. Once you identify which demographics are relevant to your research, you can make sure they’re accurately represented in your sample.
Use stratified sampling
Stratified sampling divides the population into subgroups (strata) based on shared characteristics, such as age, gender, or income. You then randomly select samples from each subgroup, improving proportional representation. This method reduces sampling errors by capturing diversity and making your sample more reflective of the overall population.
Random sampling
Random sampling helps avoid bias when picking study participants by giving everyone an equal shot at being chosen. A method like this provides a fair, unbiased sample that better represents your target group.
Replicate the study
To minimize sampling errors, researchers often employ replication strategies. These may include repeated measurements, expanding the subject pool or group size, or conducting multiple studies on the same topic. The aim of these approaches is to enhance the reliability and generalizability of research findings.
Minimize sampling errors with Prolific’s global participant pool
Generally speaking, sampling errors may be unavoidable. But with the right tools and preparation, you can lessen their impact on your study.
Prolific helps minimize your sampling errors. Our extensive pool of over 200,000 active participants in 38 countries means you can find a large number of reliable participants with ease. Choose from sample presets or build a unique sample data set based on the demographic requirements of your research population.
Start gaining more accurate results. Sign up to Prolific for free today.