Stratified vs. Cluster sampling
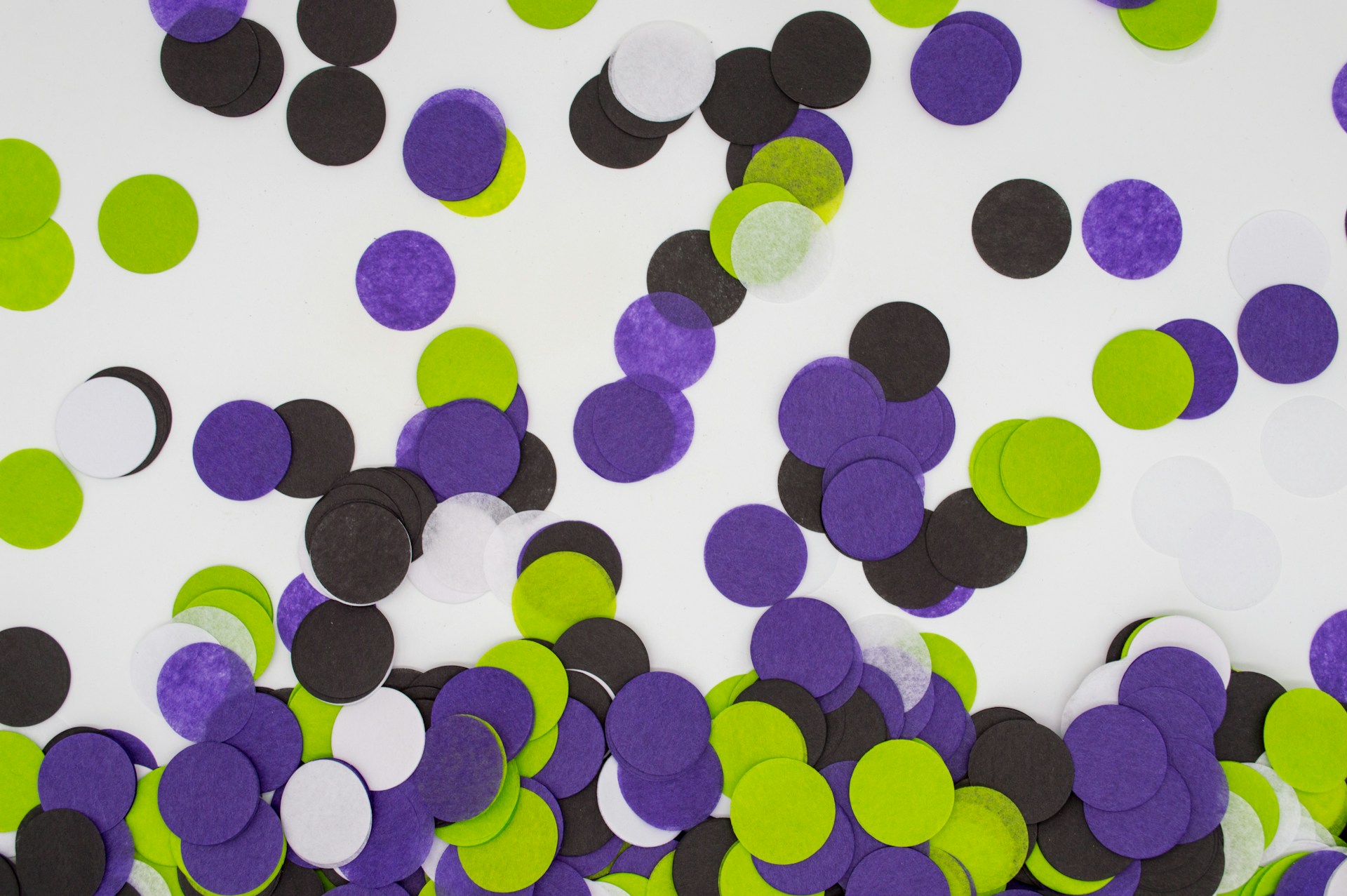
Imagine you’re a researcher searching for insights that could shape industries, policies, and even lives. The success of your study relies heavily on one factor: sampling methodology. If you make a mistake here, even the best findings might be dismissed as inaccurate or not representative.
Two important sampling methods are stratified sampling and cluster sampling. Here, we help you understand both, including their theories and their trade-offs.
Stratified vs. Cluster sampling
Both methods aim to create representative samples. However, they differ in how they define key subgroups.
What is stratified sampling?
Stratified sampling divides a population into subgroups called 'strata' based on shared traits, which can include factors like age, gender, location, or any characteristics relevant to the research.
Often, a stratum is defined by a combination of factors—for example, age (18-30), gender (male), and education (degree). By sampling within these more detailed cross-sections, you show that each demographic group is fairly represented.
This approach provides a more accurate and detailed picture of the population, such as health behaviors. The goal is for these strata to represent the overall population accurately.
Researchers then draw a random sample from each stratum, which mirrors the real population and reduces bias, leading to more reliable estimates.
What is cluster sampling?
Cluster sampling groups people by a factor, such as geographic areas, neighborhoods, or cities. Researchers then randomly select entire clusters and survey everyone within them.
This method treats existing groups as subgroups to make the sampling process simpler.
For instance, if you're studying educational outcomes across a country, you might randomly choose entire schools (clusters). You would survey all students within those schools to be more practical and save money. It's especially useful for large, spread-out populations.
The same, but different
Stratified sampling deliberately creates subgroups that represent key population segments and characteristics. Cluster sampling, on the other hand, treats naturally existing groups of people clustered together as the subgroups themselves.
What are the key differences between stratified and cluster sampling?
Stratified and cluster sampling differ in how they define and create subgroups for the final sample.
Stratified sampling | Cluster sampling |
Creates subgroups based on specific variables like demographics or behaviors. | Uses geographic areas as pre-existing clusters to sample from. |
Uses detailed data to mirror population breakdowns within these strata. | Simplifies logistics by surveying entire clusters. |
Offers precise control over the final sample composition. | May trade some representativeness for practicality and cost-effectiveness. |
Why is the distinction important?
This difference in subgroup construction affects various aspects, including logistics, costs, precision, and bias.
Stratified sampling | Cluster sampling |
Provides detailed control over sample composition. | Simplifies the sampling process by avoiding the stratum creation step. |
May involve complex logistics and data collection, but can also be simplified through techniques like crowdsourcing. | Involves lower costs and simpler logistics. |
Enhances precision but at a higher operational cost. | Can lead to higher sampling errors if the chosen clusters do not adequately represent the diversity of the overall population.
|
When to use each approach
Stratified sampling is ideal when:
- The representation of key subgroups is noteworthy.
- The research involves extensive subgroup analysis or comparisons.
Cluster sampling is better for:
- Large-scale surveys on a regional, national, or international level.
- Studies focused on geographic trends or neighborhood dynamics.
- Situations where operational efficiency and cost constraints outweigh the need for detailed subgroup representation.
Combining both methods
Often, the best sampling strategy isn’t a simple choice between stratified or cluster sampling. Instead, a combined approach, known as stratified cluster sampling, can be used.
In this method, the population is first divided into strata based on key variables (like age, gender, or education). Then, within each stratum, researchers randomly select geographic clusters (such as neighborhoods or districts) and sample all units within those clusters.
An approach like this allows researchers to achieve subgroup representation through stratification, while also benefiting from the cost and logistical efficiency of clustering.
It’s more useful, however, when geographic clusters naturally align with the variables of interest. In situations where a variable like education doesn’t geographically cluster, this approach may not be appropriate.
Possible real-world example: national health survey
Consider a national health survey. You want to understand health behaviors across different regions and age groups.
Using stratified sampling, you first divide the population into distinct age groups. Then, within each age group, you can apply cluster sampling by selecting specific regions or neighborhoods to survey. Instead of surveying every individual in the country, you survey a random sample of clusters within each age group.
With this approach, you can capture the diversity of each age group, while making the survey process more efficient by focusing on specific regions rather than the entire population.
Pros and cons of stratified and cluster sampling
To choose the best sampling strategy, you need to evaluate the advantages and disadvantages of each method.
Stratified sampling
Advantages of stratified sampling | Disadvantages of stratified sampling |
Greater accuracy by controlling the composition of the sample. | More operationally intensive and time-resource-heavy. |
Guaranteed inclusion of all relevant subgroups. | Requires access to comprehensive data on population subgroups. |
Ability to calculate estimates and insights for individual strata. | Potential for sampling bias if strata are defined incorrectly. |
Generally lower sampling errors by reducing variability within strata. |
Cluster sampling
Advantages of cluster sampling | Disadvantages of cluster sampling |
Simpler logistics and lower costs compared to stratification. | Less accuracy and higher potential for sampling errors. |
Efficient for wide geographic sampling. | No guarantee all relevant subgroups will be represented. |
Captures influence of ‘cluster’ effects on concentrated populations. | Risk of unrepresentative findings if selected clusters are not representative. |
Viable for surveying remote, hard-to-reach segments. |
Practical applications and examples
What does stratified and cluster sampling look like in action? Here are some practical applications to show when and how to use these sampling methods.
Educational survey across a country (stratified)
For a national educational survey, stratified sampling would involve dividing the population into subgroups, such as by age, region, or education level, and then sampling within each group. This ensures that each subgroup is proportionally represented, giving a detailed and accurate view of educational trends across the country.
Health study in a city (cluster)
If you're conducting a health study in a city, cluster sampling would involve selecting specific neighborhoods or districts and surveying all residents within those areas. Using this method simplifies the logistics of data collection while still capturing health trends, though care must be taken to choose representative clusters.
National health survey (both)
In a national health survey, you could use stratified sampling to divide the population into key groups, like age or income brackets, achieving proportional representation. Then, within each group, you'd apply cluster sampling, selecting specific neighborhoods to survey, reducing costs while maintaining diversity across demographic groups.
Guaranteeing representative samples
Both stratified and cluster sampling aim to derive samples that should accurately represent the larger population. However, the success of either method depends on careful planning and execution.
For stratified sampling | For cluster sampling |
Ensure strata are defined based on variables that reflect the population's diversity. | Select clusters based on key demographic or geographic variables to better capture the diversity of the population.
|
Use accurate, up-to-date data to create strata. | Be aware of potential cluster effects that could influence results. |
Randomly sample within each stratum to avoid bias. | Consider combining with stratification for more balanced sampling. |
Challenges and considerations
No sampling method is perfect. Both stratified and cluster sampling have their challenges.
For stratified sampling:
- Defining strata correctly is crucial to your results. If you choose the wrong variables, your sample might not be representative.
- Collecting detailed data to create strata can be time-consuming and costly.
- Ensuring random sampling within each stratum is essential to avoid bias.
For cluster sampling:
- Choosing clusters that accurately reflect the overall population is necessary. If the selected clusters don't capture the diversity of the population, your results may be biased or skewed, as they won't represent the full range of characteristics present in the population.
- Larger sample sizes might be needed to achieve the same level of accuracy as stratified sampling because cluster sampling often introduces more variability within clusters. Since clusters may not represent the full diversity of the population, more clusters or participants could be needed to reduce sampling error and ensure reliable results.
- Understanding and accounting for cluster effects is important to ensure accurate results.
Summary: stratified vs. cluster sampling
Both stratified and cluster sampling are powerful methods for creating representative samples. They also yield high-quality insights. Each method has its strengths and weaknesses, and the best choice depends on your research needs.
Understanding the pros and cons of each approach means you can make informed decisions. You can choose the method, or a hybrid, that best meets your study's objectives. Careful planning and execution of your sampling strategy will help you gather strong, representative data. What you’ll get from that are useful insights and impactful research results.
Learn more about sampling with our complete guide to representative sampling