What is stratified sampling?
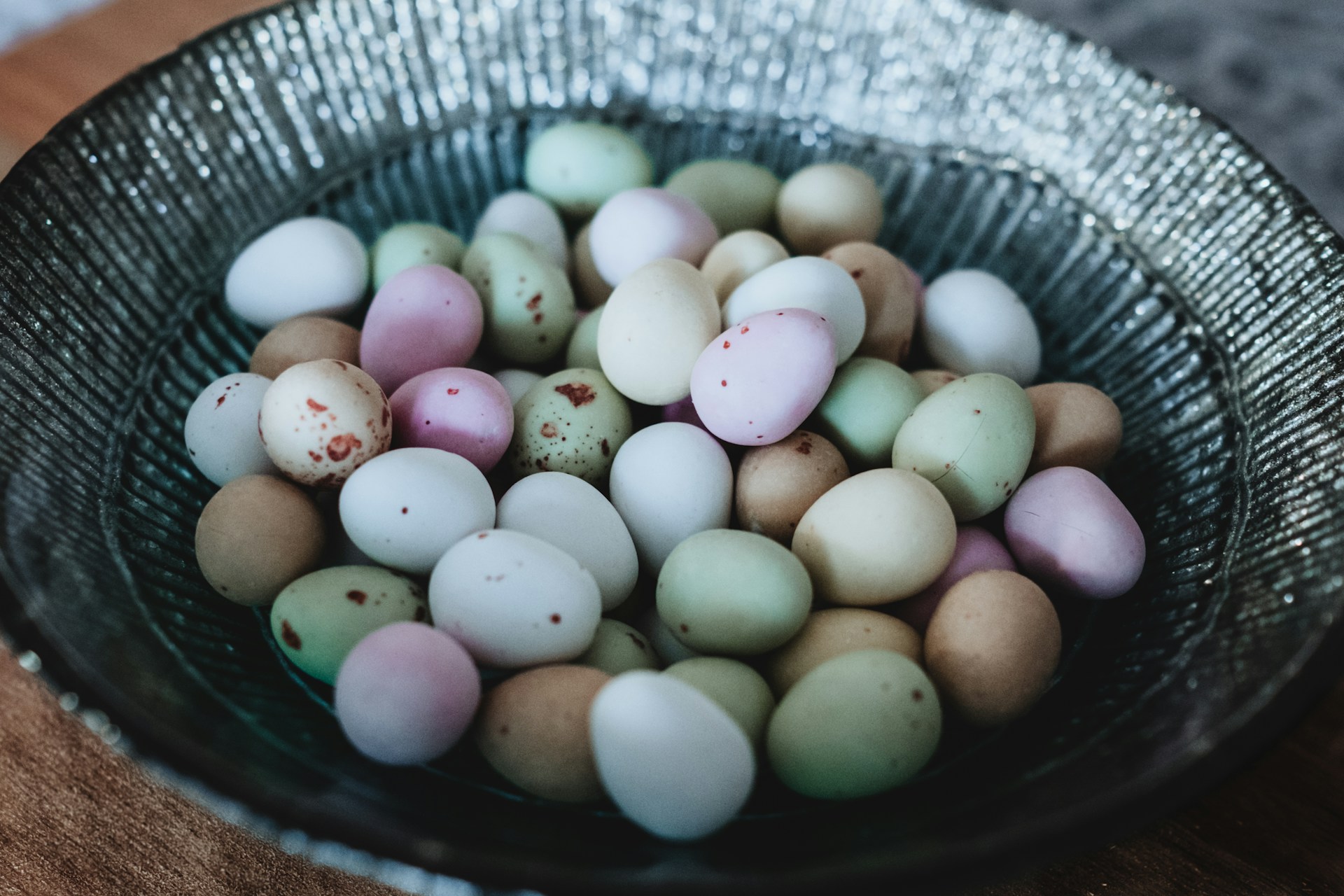
When studying any population, getting a sample that accurately reflects its diversity is fundamental to your research. However, using something like convenience sampling risks missing important subgroup differences.
These demographic differences in the sample may be key predictors of study effects, and without adequately representing these demographics, there is a risk of overlooking important findings.
This is where stratified sampling comes in handy. It deliberately segments the population into distinct strata. Based on important demographic variables, it samples from each stratum proportionally to the population as a whole.
Here, we have all you need to know about stratified sampling, including how it works and when you might need it in your research.
What is stratified sampling?
Stratified sampling is a type of probability sampling. The target population is first split into separate, non-overlapping subgroups. We call these subgroups strata. Based on shared traits or characteristics, these segmenting variables are used to define the strata and can include demographics like:
- Age groups (e.g. 18-24, 25-34, 35-49, etc.)
- Gender identities
- Income tiers
- Education levels
- Ethnic and racial demographics
- Geographic regions, densities (urban and rural), etc.
The key requirement is that each stratum reflects a subgroup's true proportion in the larger population being studied. For instance, if adults aged 65 and older make up 18% of the population, they must also represent 18% of the sample.
We create strata definitions by analyzing verified data sources, such as government census databases. These databases measure the existence and makeup of subgroups in the population. Researchers then use standard probability sampling techniques, such as simple random sampling, to randomly select participants within each stratum.
The final sample combines the subsamples from each stratum. It’s designed to capture every subgroup in the population with precise, statistically accurate representation.
Examples of stratified sampling
Let's examine a few examples to show how stratified sampling works.
Consumer product testing
Imagine a large food company wants to test a new line of snacks nationwide. To build a stratified sample accurately reflecting key consumer demographics, they could define their strata as follows:
- Gender (male, female)
- Age brackets (13-17, 18-24, 25-34, 35-54, 55-64, 65-plus)
- Household income Tiers (under £25K, £25K-£50K, £50K-£100K, over £100K)
- Geographic region (north, south, midlands, northeast)
- Household Composition (single, couple, family with kids, multi-generational)
The researchers would then work with trusted demographic data providers to find the real national percentages for each segment.
For example, they would find that 13.2% of the population is aged 55-64. They'd also find that 24.6% of households are single-person and 18.9% of the population lives in the Northeast.
Based on those percentages, they would set specific sample size targets for each stratum and fill them by sampling participants randomly within each stratum. In this case, the number of strata would be based on the possible combinations of sub-strata (e.g., age, gender, income, and region).
For instance, a single stratum might represent male, aged 13-17, with income under £35K, living in the North, and single. Given the complexity, there could be hundreds of different strata—each requiring its own sampling target.
If the national sample goal is 4,000 people, the 55-64 age group alone may need around 530 people (13.2% of 4,000).
Healthcare effectiveness study
Now, let's consider an example in healthcare research. It's about studying the impacts and effectiveness of a newly approved medication. In this case, researchers may stratify their participant sample by:
- Age range (18-34, 35-44, 45-54, 55-64, 65-plus)
- Gender identity (male, female, non-binary, other)
- Geographic region (north, south, midlands, northeast)
- Insurance coverage type (private, public, uninsured)
- Pre-existing conditions (none, obesity, diabetes, heart disease, etc.)
- Dosage level of medication (low, medium, high)
This plan accounts for age and region. It also includes clinical variables that could influence the impact of the medication. The plan guarantees that no disadvantaged subgroups are overlooked. Researchers would work carefully with health data sources and use them to measure distributions across these groups.
During sampling, patients would be randomly selected from each stratum. For example, the goal might be to ensure that 23% of the total sample consists of uninsured patients under age 35 in the Midlands with obesity. Taking this approach enhances the validity of statistical conclusions about the medication's effects across different subpopulations.
Academic sociological study
Let's consider how stratification could improve sampling for academic studies. These studies explore fields like sociology, political science, and economics. Researchers may want to study attitudes, beliefs, and behaviors related to a nationally relevant societal issue, such as educational inequality.
Their stratified sample design may include strata based on variables like:
- Age range (18-24, 25-34, 35-44, 45-54, 55-plus)
- Gender identity (male, female, non-binary, other)
- Highest level of education (high school, college, postgraduate, other)
- Ethnic racial demographic background (White, Black, Asian, Mixed, Other)
- Annual household income level (under £25K, £25K-£50K, £50K-£100K, over £100K)
- Geographic region (north, south, midlands, northeast)
- Political party affiliation (conservative, liberal, independent, other)
This intricate stratification accounts for demographic subgroups with various sociological variables. It also considers behavioral and ideological factors that shape perspectives on the issue being studied.
By using detailed census data and population estimates, strata definitions are precisely engineered. Randomized sampling then occurs independently within each stratum. For example, you might focus on Pakistani males aged 40-49 in West London with incomes under £50K who lean conservative.
The final sample is stratified and combines weighted subsamples. This method aims to reflect the diverse identities and demographics of the population, enhancing the accuracy of insights for each subgroup.
Advantages and disadvantages of stratified sampling
Like any advanced sampling method, stratified sampling has advantages and disadvantages. These should be carefully considered in relation to the specific research goals and practical constraints, such as time, budget, or available data.
Key advantages:
- Greater accuracy and efficiency in results, as sampling variance is reduced within each stratum.
- Guaranteed proportional representation of all definable subgroups of interest within the population.
- Stratified sampling supports more detailed analyses, such as regression models or factor analysis, by allowing researchers to examine relationships between multiple variables.
- It focuses sampling only on demographics that are most relevant to the research subject.
- Isolates potential confounding factors by segregating the entire population into individual strata.
Potential limitations:
- Labor-intensive process requiring extensive upfront coordination with data providers on stratification criteria.
- It’s more complex and incurs higher costs due to the need for detailed data collection and management. You need to sample within each stratum separately.
- The potential for improper or skewed strata definitions can introduce systematic biases if not carefully designed.
- Less flexible than unstratified sampling at retroactively shifting or exploring differences between specific demographics.
- Access to accurate and reliable demographic data is necessary. These benchmarks, such as census data or verified population statistics, are needed to define valid, mutually exclusive strata.
When implemented by experienced researchers, stratified sampling provides unmatched capabilities for generating representative, demographically-attuned participant samples. This makes research more representative. It also improves the validity of conclusions for studies exploring topics known to be influenced by subgroup dynamics.
However, the upfront procedures are complex, and the operations are demanding. They may be too much for some research scenarios. In those scenarios, simple techniques are enough to get initial insights, which are less certain but still useful.
When to use stratified sampling
Stratified sampling has unique advantages. However, it has tradeoffs in cost or complexity, and this method is best used for specific research goals and contexts.
Stratified sampling is generally considered ideal when:
- Understanding differences between groups in responses is a key research priority.
- There are empirical foundations or strong hypotheses suggesting that demographics such as age, income, and ethnicity greatly influence the topics being studied.
- Proper inclusion and intentional oversampling of underrepresented minority groups or low-prevalence subpopulations is imperative for representativeness.
- You can use reliable, high-quality demographic benchmarks. They accurately define strata.
Conversely, basic random sampling techniques may suffice when:
- The research goal is to make rough population estimates. They will be at a high level and cover the whole population. They will not need in-depth subgroup breakouts.
- No specific demographics are thought to directly impact the variables.
- Limited project budgets, timelines, or resourcing constraints prohibit the upfront complexities involved in rigorous stratified sampling protocols.
Stratified sampling is an advanced probability technique. It’s unparalleled in matching sample composition with true demographic diversity. This is especially true when done with proper statistical care. For research where demographic identity and communal subgroup representation underpin resonant conclusions, the value stratification provides is unmatched.
Summary: stratification
Stratified sampling captures audience diversity from the ground up. It reflects a core idea: populations are not monoliths. They are intersections of identities shaping lived realities. For important research, you need real representation and community-aligned insights. Stratification provides the demographic segmentation needed to credibly illuminate those human truths.
Learn more about sampling techniques in our complete guide to representative sampling